Wolfram's Theory Exposes the Inherent Ceiling of Artificial Intelligence
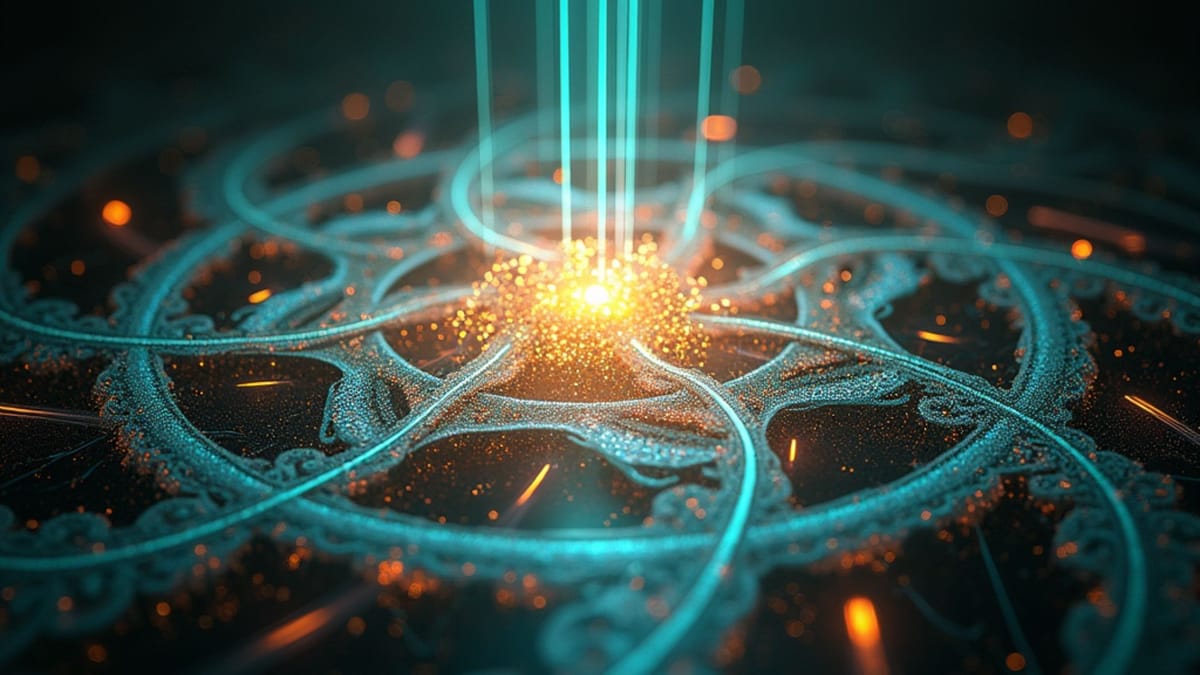
"If we could predict what an AI system would output without running it, we wouldn't need to run the AI at all," explains Stephen Wolfram, applying his decades-old theory of computational irreducibility to expose fundamental limitations in even the most advanced artificial intelligence systems.
This insight represents a significant counter-narrative to typical AI enthusiasm, writes End of Miles, offering a theoretical framework for understanding why achieving artificial general intelligence may be more complex than many technologists anticipate.
The Paradox of AI Capabilities
Wolfram, creator of Mathematica and Wolfram Alpha, argues that modern AI systems demonstrate an interesting paradox: they excel at tasks humans find intuitive while struggling with problems requiring deep computational work.
"What AI systems are good at is the stuff that humans are also good at—the human reasoning types of things. My guess is they're good at that because they basically work more or less the same way that our brains work," Wolfram explains. "They are extrapolating, generalizing in ways that seem sensible to us because they're doing it the same way we do it." Stephen Wolfram
The computational theorist points to AI's impressive ability to recognize patterns humans miss, like subtle facial aging markers or semantic patterns in language. However, these capabilities come with inherent boundaries that his concept of computational irreducibility helps explain.
Nature's Complexity vs. AI's Simplicity
Computational irreducibility, a concept Wolfram introduced in his 2002 book "A New Kind of Science," suggests that some systems cannot be simplified or predicted through shortcuts—they must be explicitly run to determine their outcome.
"If you say 'solve this computationally irreducible problem—figure out what's going to happen in a three-body gravitational system,' that's probably an example of computational irreducibility. AI does pretty criminally with that. It does things which are kind of intuitively sensible, but when it comes to the details of the irreducible computation, it doesn't do very well." The Wolfram Alpha founder
The distinction Wolfram draws is crucial: human engineering traditionally avoids computational irreducibility, while nature embraces it. AI systems, trained on human-created patterns, consequently share our limitations in handling deeply complex systems.
Redefining AI's Value Proposition
Rather than viewing these limitations as a failure, the computational pioneer suggests they point toward AI's true value—operating within the same psychological framework as humans but with different pattern recognition capabilities.
"What the AI will do well potentially is say 'based on the 4 million papers that humans have written, this is something humans might care about,'" notes the scientist. This capability to identify which computationally irreducible problems might interest humans represents a different but valuable contribution.
Wolfram's perspective offers a critical theoretical foundation for understanding why even exponential improvements in AI technology won't necessarily lead to systems that can solve our hardest scientific problems. The computational limits he identified decades ago may prove to be the ceiling against which artificial intelligence ultimately bumps—not because of engineering failures, but because of the irreducible nature of computation itself.