The Surprising Bottleneck Holding Back Artificial General Intelligence
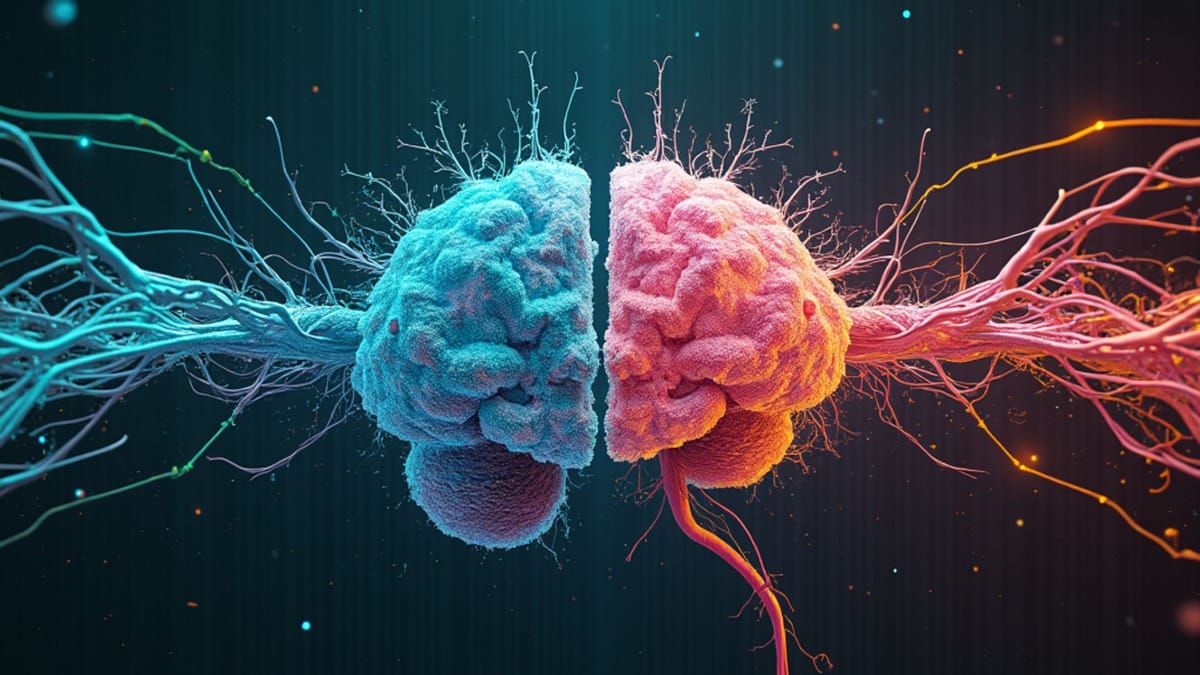
"AI systems can solve graduate-level physics problems but can't reliably navigate a simple text-based game without getting stuck in ridiculous loops. This disconnect isn't just an amusing quirk—it may be the fundamental bottleneck delaying truly transformative AI," explains Ege Erdil, a researcher at Epoch AI, during a candid discussion with colleague Matthew Barnett on diverging AGI timelines.
End of Miles reports that this critical insight into AI development challenges traditional narratives about how and when artificial general intelligence might transform the economy.
The Moravec Paradox in action
While today's most advanced AI systems demonstrate remarkable capabilities in domains like mathematical reasoning and competitive programming, they struggle profoundly with tasks most humans consider trivial. This pattern reflects what's known as Moravec's Paradox—the counterintuitive observation that high-level reasoning can be easier to automate than common sense abilities.
"We haven't seen anywhere near as rapid progress on the capabilities that would actually unlock a lot of economic value. I think it's much more important to have agency and ability to sort of adapt plans to changing circumstances and just do simple tasks in a reasonably competent way." Ege Erdil, Epoch AI researcher
Both Epoch researchers noted that the most economically valuable capabilities are not necessarily the ones that appear most impressive to humans. The Stanford expert emphasized that tasks requiring advanced reasoning and specialized knowledge—like solving mathematical theorems—represent a tiny fraction of the economy, while mundane abilities to function as a competent agent represent the bulk of valuable human labor.
The hidden complexity of 'simple' tasks
The researchers highlighted examples showing how AI systems fail at tasks that appear simple. In one example, when given a basic command-line environment with files to search, even sophisticated reasoning models fail to develop efficient search strategies—approaches that would be obvious to most humans.
"They will often say 'oh the game is broken' instead of saying 'I made a mistake, I have to correct my model of this situation.' It just seems so bizarre that you would fail on such a simple task of reasoning." Erdil describing AI failures in game environments
These gaps suggest that the capabilities humans take for granted—our ability to plan, adapt, and function competently in changing environments—may be evolutionary achievements that are exceptionally difficult to replicate through current AI methods.
Economic implications
Both AI experts argue that the timeline to transformative AI depends more on overcoming these agency limitations than on further improvements in reasoning capabilities. While acknowledging uncertainty, Erdil suggested this could put truly transformative economic impacts decades away rather than years.
Barnett, who holds more optimistic timelines, nevertheless agreed that agency remains a critical challenge, though he questioned whether it might be solved through scaling and transfer learning sooner than his colleague expects.
This perspective challenges popular narratives around AI progress that focus on benchmark achievements in reasoning tasks. The agency gap suggests that transformative economic impact may require breakthroughs in capabilities that are currently receiving less attention than flashier reasoning demonstrations.