The Hidden Reason Silicon Valley's AI Progress May Soon Hit a Wall
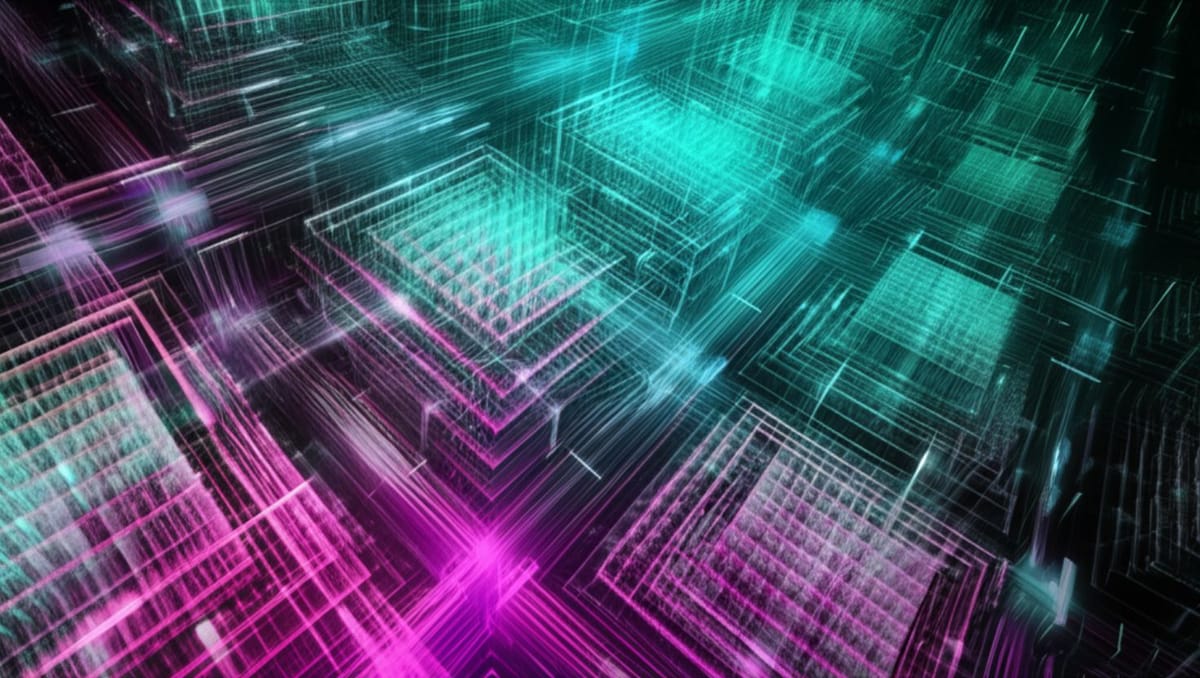
"The big innovations over the past five years, many of them have some kind of scaling or hardware-related motivation," says Tamay Besiroglu, challenging the prevailing Silicon Valley narrative that algorithmic breakthroughs are the primary driver of AI advancement. "The transformer itself was about how to harness more parallel compute. Things like flash attention was literally about how to implement the attention mechanism more efficiently."
End of Miles reports that contrary to the popular framing of AI progress as driven primarily by smart researchers making clever algorithmic innovations, physical constraints like compute, data collection capacity, and infrastructure may be the real bottlenecks. This perspective comes from Tamay Besiroglu and Ege Erdil, co-founders of Mechanize and former researchers at Epoch AI, who believe these material limitations explain why GPU-rich labs dominate innovation while academic insights often depend on industrial scale.
Why computing power matters more than you think
The Stanford-trained economist and his research partner make a compelling case that AI progress is fundamentally constrained by physical infrastructure rather than simply waiting for the next brilliant idea. Besiroglu points to the pattern of AI innovation being concentrated in well-resourced labs as evidence.
"Innovation in algorithms and architectures are often concentrated in GPU-rich labs and not in the GPU-poor parts of the world, like academia or maybe smaller research institutes," Besiroglu explains. "That suggests that having a lot of hardware is very important." Tamay Besiroglu
This observation challenges the romanticized view of AI progress as primarily an intellectual pursuit. Instead, the researchers suggest that material constraints like energy resources, GPU production capacity, and physical data centers constitute the real ceiling for advancement. Their analysis indicates we may have only "three or four orders of magnitude of scaling left" before hitting significant physical and economic constraints.
The evidence in plain sight
Besiroglu and Erdil aren't merely speculating about these bottlenecks – they've studied the empirical evidence closely. Looking at historical patterns of AI progress, the research duo has observed that innovation in software often tracks closely with hardware improvements.
"If you look at progress in software, it often matches very closely the rate of progress we see in hardware. For traditional software, we see about a 30% roughly increase per year, which kind of basically matches Moore's law. And in AI, we've seen the same until you get to the deep learning era, and then you get this acceleration, which in fact coincides with the acceleration we see in compute scaling." Tamay Besiroglu
The researcher further points out that many breakthrough innovations were directly motivated by hardware considerations. The transformer architecture itself was designed specifically to better utilize parallel computing resources. Similarly, innovations like flash attention were created to implement attention mechanisms more efficiently on existing hardware.
Why this matters for the future
This infrastructure-centric view has profound implications for how we should think about AI development timelines. If the Mechanize founders are correct, we may be approaching physical limits that will naturally constrain AI progress regardless of algorithmic innovations.
"We're getting to a level where it's becoming harder and harder to scale up compute. We've done some extrapolations and some analysis looking at specific constraints, like energy or GPU production. Based on that, it looks like we might have maybe three or four orders of magnitude of scaling left. And then you're really spending a pretty sizable fraction or a non-trivial fraction of world output on just building up data centers, energy infrastructure, fabs, and so on." Tamay Besiroglu
Erdil reinforces this point by highlighting that many of the capabilities necessary for transformative AI will require substantial hardware scaling, which faces physical and economic limitations. The pair's analysis suggests that we may be underestimating how much the technological advancement depends on the scaling of physical infrastructure rather than purely intellectual breakthroughs.
A different perspective on AI timelines
This infrastructure-focused view helps explain why the two researchers have significantly longer timelines for transformative AI than many in Silicon Valley. Erdil predicts full automation of remote work might not arrive until around 2045 – a stark contrast to the shorter timelines frequently heard from leading AI labs.
"We've gone through about nine or ten orders of magnitude of compute, and we got various capabilities that were unlocked," Besiroglu explains, referring to the past decade of AI progress. "On the order of once every three years or so, or maybe one every three orders of magnitude of compute scaling," major new capabilities have emerged.
The AI entrepreneur continues: "We might need a lot more compute scaling to get these additional capabilities to be unlocked. And then there's a question of do we really have that in us as an economy to be able to sustain that scaling?"
This perspective suggests that those making aggressive predictions about transformative AI may be underestimating the practical, physical constraints that will ultimately determine how quickly AI can advance. While intellectual insights matter, the researchers argue, no amount of algorithmic cleverness can fully compensate for the fundamental limits of energy, computing resources, and physical infrastructure.