The Bigger the Better: Biological AI Models Show Size Matters in Cell Analysis
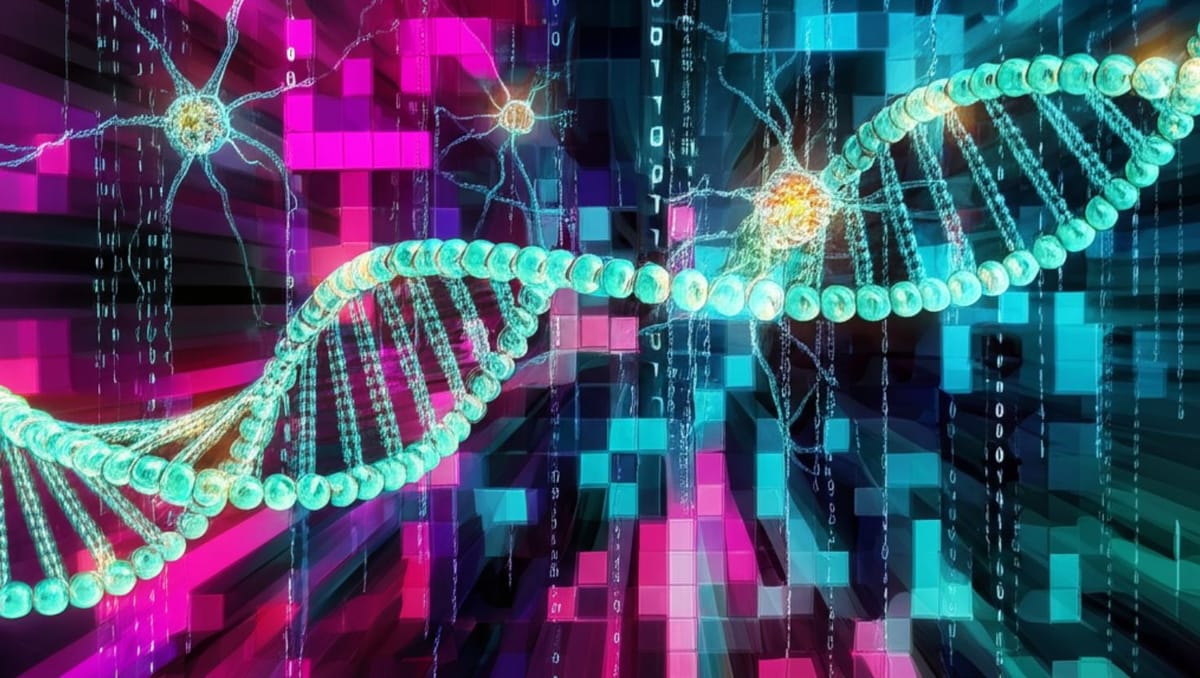
"With more data and compute, biological language models will keep getting better, opening the door to increasingly sophisticated and generalizable tools for biological discovery," says Yale Assistant Professor David van Dijk, revealing a breakthrough finding that could transform how scientists approach computational biology.
End of Miles reports that researchers from Yale University and Google Research have discovered that language models trained on biological data follow the same predictable scaling laws seen in general artificial intelligence systems.
Size matters in biological AI
The discovery came as part of the team's work on Cell2Sentence-Scale (C2S-Scale), a family of AI models designed to "read" and "write" biological data at the single-cell level. The researchers found that as they increased the size of these models from 410 million to 27 billion parameters, performance consistently improved across various biological tasks.
"A central finding of our work is that biological language models follow clear scaling laws — performance improves predictably as model size increases." David van Dijk and Bryan Perozzi
This pattern mirrors what AI researchers have observed in general-purpose large language models like those powering ChatGPT or Google's Gemini, where bigger models consistently outperform smaller ones. The Yale professor and his team documented these improvements across both predictive tasks (measured by semantic similarity) and generative tasks (measured by gene expression overlap).
The biological scaling trajectory
The discovery wasn't just academic—it came with measurable benefits. The research scientist from Google noted significant performance gains in critical biological applications when scaling up model size.
"For dataset interpretation, we observed consistent gains in semantic similarity scores when scaling model size in the parameter-efficient regime. With full fine-tuning, gene overlap percentage in tissue generation significantly improved as model capacity increased to 27 billion parameters." Bryan Perozzi
This finding carries profound implications for computational biology. Just as general AI has seen capabilities explode with model scaling, the field of biological modeling may be on a similar trajectory—suggesting the coming years could bring dramatic improvements as larger models are built.
Why scaling laws matter for medicine
The scaling phenomenon has particular significance for medical research. The C2S-Scale models can already perform sophisticated tasks like predicting how cells will respond to cancer drugs or generating realistic "virtual cells" for in silico experimentation.
If these capabilities follow the same scaling trajectory seen in other AI domains, future biological language models could revolutionize drug discovery, disease modeling, and precision medicine approaches. The Yale-Google collaboration suggests that computational biology stands at the beginning of an exponential improvement curve similar to what has transformed natural language processing.
The biological AI systems developed by the research team transform gene expression data into text sequences called "cell sentences," making it possible to apply natural language processing techniques to complex biological problems. By demonstrating that these models follow predictable scaling laws, van Dijk and his collaborators have established a roadmap for future development in the field.
"With more data and compute, biological LLMs will keep getting better, opening the door to increasingly sophisticated and generalizable tools for biological discovery." The research team
All models from the project are being released as open-source, allowing researchers worldwide to build on these findings and accelerate progress in computational biology.