Semantic Richness—Not Algorithms—Drives AI Innovation, MIT Study Finds
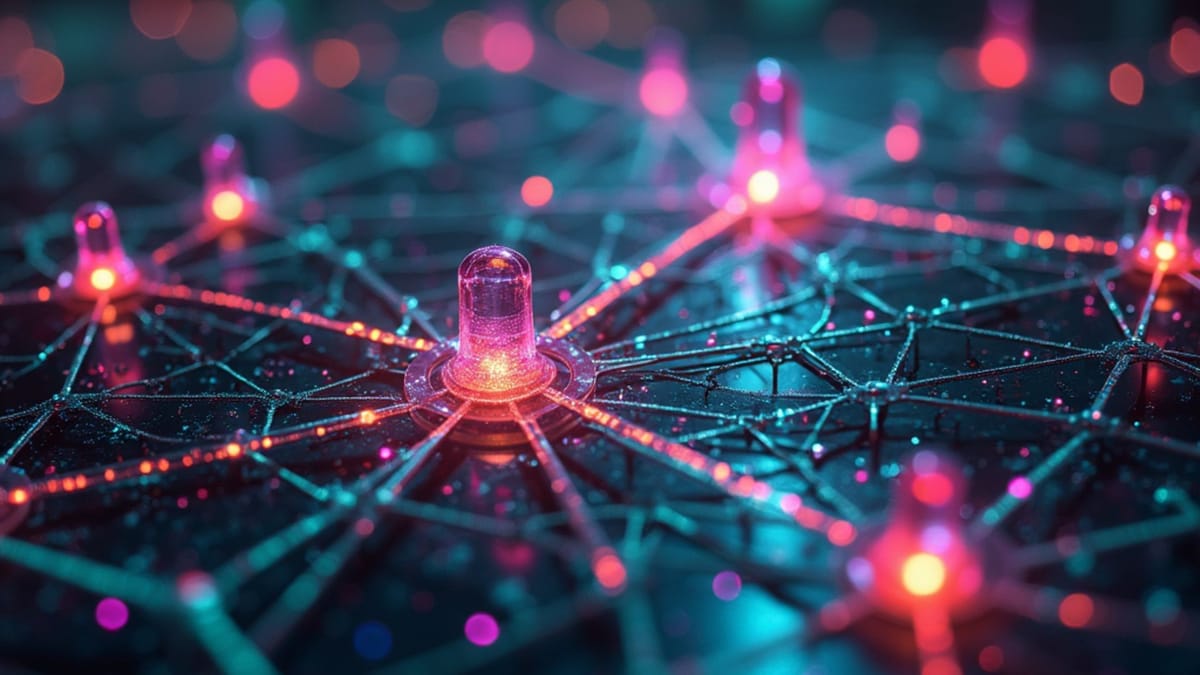
The continual innovation in advanced AI systems isn't primarily driven by increasingly complex algorithms but rather by the richness of semantic meaning that emerges during the reasoning process, according to groundbreaking research from MIT.
End of Miles reports on this paradigm-shifting finding, which challenges the conventional understanding of how artificial intelligence generates new insights and discoveries.
The meaning behind the machine
Professor Markus J. Buehler of the Massachusetts Institute of Technology has identified a subtle yet persistent dominance of semantic entropy (the diversity of meaning) over structural entropy (the complexity of connections) in reasoning-based AI systems. This previously unrecognized dynamic appears to be fundamental to continuous innovation.
"Our central finding reveals that continuous innovation in agentic reasoning systems arises fundamentally from entropy dynamics, specifically a subtle yet persistent dominance of semantic entropy over structural entropy," Buehler explains in his research published on March 25.
The MIT scientist and his team analyzed the evolution of AI-generated knowledge graphs through numerous reasoning cycles. By rigorously measuring both structural entropy (using Von Neumann graph entropy) and semantic entropy (based on embedding diversity), they discovered that semantic entropy consistently remains higher throughout the evolution of the system.
Why this matters for AI development
This discovery fundamentally challenges how we understand machine intelligence and could redirect approaches to developing more creative AI systems. Rather than focusing solely on architectural improvements, Buehler's work suggests that semantic richness may be the key driver of innovation.
"The reason artificial reasoning systems remain continuously creative and innovative may be because they constantly explore a very rich, diverse, and somewhat chaotic space of possible meanings. In contrast, the actual connections the system forms, its explicit reasoning structure, are more ordered and constrained." Buehler notes in the study.
The research demonstrates that approximately 12% of connections in successful AI reasoning systems link semantically distant concepts—a ratio that remains stable throughout the evolution of the system. These "surprising edges" appear to be critical for sustained discovery and innovation.
Universal principles of discovery
Perhaps most intriguing is the parallel Buehler draws between AI reasoning systems and natural complex systems like biological networks and physical phase transitions. The research suggests that the balance between semantic richness and structural organization may represent a universal principle governing adaptability and innovation.
"Because the system always has more meaningful ideas available to explore than it explicitly incorporates into its structure, it can continuously discover and create unexpected, novel relationships. This ongoing imbalance between rich semantic possibilities and more structured connections is what fuels sustained creativity and innovation." The MIT researcher explains.
Based on these insights, Buehler has proposed a reinforcement learning framework that could potentially maximize continuous semantic discovery in future AI systems, explicitly targeting this balance between semantic diversity and structural coherence.
From theory to practice
For AI developers, these findings suggest a potential shift in focus from purely architectural improvements toward methods that enhance semantic richness. The Critical Discovery Parameter (D) introduced in the research could become a standard metric for evaluating an AI system's innovative potential.
As companies race to develop increasingly capable AI systems, this research offers a new lens through which to understand what drives continuous discovery and creativity—not just the complexity of the algorithm, but the richness of meaning the system can explore.