NYU AI Architect: AI Adoption Requires Behavior Change, Not Tech Skills
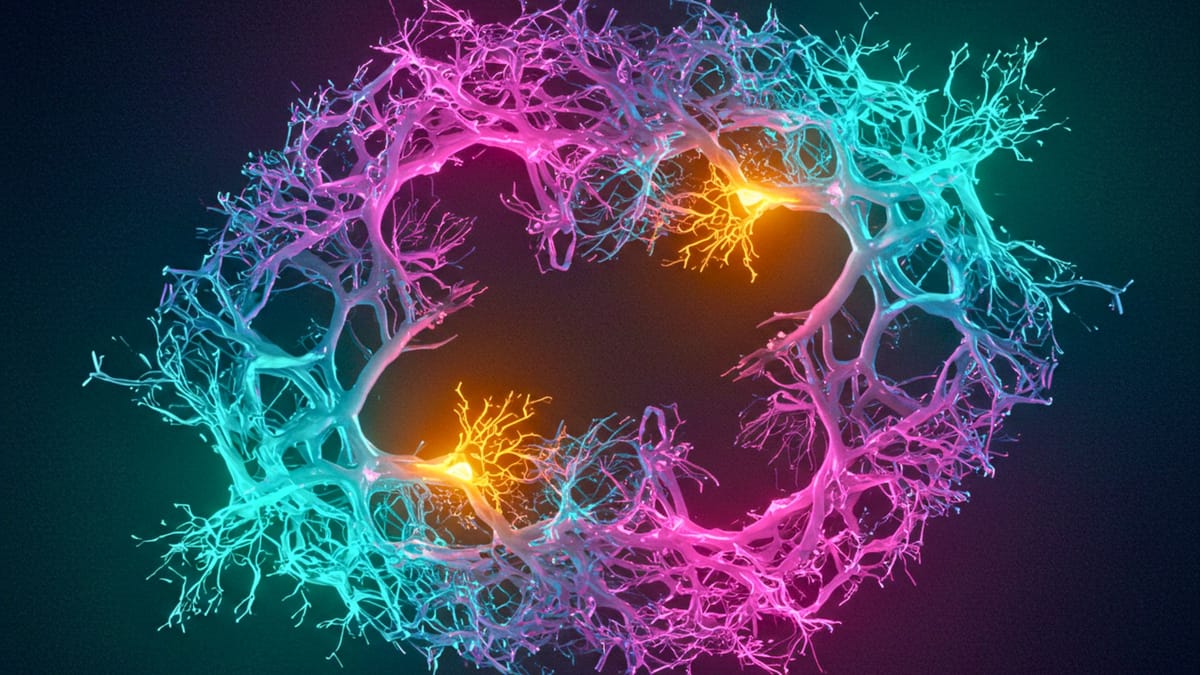
"This is a technology that doesn't really act like a normal technology. This is sort of a software that acts more like a human than software," says Connor Grennan, describing why organizations struggle with AI adoption despite significant investments. The chief AI architect at NYU's Stern School of Business has identified a fundamental misconception at the heart of most failed AI implementations: treating AI adoption as a technical skills challenge rather than a behavioral change initiative.
End of Miles reports that Grennan's perspective challenges conventional wisdom in enterprise AI implementation, potentially explaining why adoption rates remain surprisingly low despite widespread access to tools like ChatGPT and Microsoft Copilot.
Why AI differs from traditional technology rollouts
According to Grennan, organizations typically approach AI implementation like any other digital transformation—teaching people to use new software, following a learning curve, and eventually replacing old systems. This approach worked for previous technologies but fails dramatically with generative AI.
"Usually when you're doing a digital transformation of some kind, you're going from some old system up to Salesforce or CRM or moving over to Excel. The way that you do that usually is to teach people how to use that software. That's how everybody was handling something like generative AI, ChatGPT, or Microsoft Copilot. And it turned out that didn't work, which is why adoption rates tend to be very, very low." Connor Grennan, NYU Stern School of Business
The NYU expert instead proposes a radically different mental model for understanding generative AI adoption. Rather than comparing AI to traditional software, Grennan suggests we view it more like exercise equipment—a tool that requires behavioral change to deliver value.
"It's actually much more like a treadmill, where it's not actually about learning how to use this treadmill. It's actually just about the behavior. You could put a treadmill in every house in America, but you're not going to see heart disease really lower. It doesn't really matter about the technology as much as it matters about the behavior." Grennan
The unique nature of generative AI
The behavioral scientist emphasizes that unlike previous technologies, generative AI doesn't require extensive technical knowledge or specialized skills. The barrier isn't understanding the technology—it's changing how people work and think.
"This technology like generative AI doesn't really require you to learn anything," explains the AI architect. "There's not really better ways to prompt, and there's not really 'oh, you need to understand AI or machine learning.' You really just need to have a conversation with it."
This fundamental difference, he argues, is why conventional approaches like standardized use cases and technical training fail to drive adoption. The human brain naturally seeks templates and defined applications for tools, but this approach limits AI's transformative potential.
Why use cases fail to drive meaningful adoption
Grennan's experience with enterprise organizations revealed a counterintuitive pattern: training workers with pre-defined use cases initially generates enthusiasm but rarely leads to sustained adoption or organizational transformation.
"I would go into a big healthcare organization, and they would say, 'Bring us the top 10 use cases.' I would Google 10 use cases, say 'Here's how you use it,' and we'd walk out of that workshop. Everybody would be super excited. Then I would check back in with them maybe a month later, and they're like, 'Oh my gosh, amazing, those use cases are tremendous.' But my reaction would always be 'But I didn't know anything about healthcare. I Googled those use cases.'" The NYU AI specialist
The problem, according to Grennan, is psychological. "The brain loves templates," he explains. "We love to sort of understand, 'Here's the tool. What do I use it for?' Your brain wants to pull this thing off the shelf to use it in those specific moments instead of using this to augment everything you do."
A new framework for AI transformation
Instead of focusing on specific applications, the AI architect advocates for a comprehensive approach that addresses underlying psychological barriers and creates behavioral change. This requires understanding how the brain processes new tools and creating frameworks that encourage integration across all workflows.
"What I use instead of use cases is trying to break down what the brain is doing, why the brain is doing it, and a whole new way of thinking so that people can come up with their own use cases," Grennan explains.
This perspective has significant implications for how organizations approach AI implementation. Rather than focusing exclusively on training specific departments or identifying use cases, Grennan recommends starting with senior leadership and working to transform organizational culture.
"You have to train senior leaders first for a couple of reasons. First of all, senior leadership has to understand what this is. I think too often they don't even really understand how to explain this to clients or how to sort of understand how this is going to impact every department. So you have to do the same training with leaders so they understand how they'll use it in their workday." Connor Grennan
By reframing AI adoption as behavioral transformation rather than technology implementation, organizations may finally unlock the productivity gains that have thus far remained elusive despite billions in AI investments.