New AI Weather Model Could Revolutionize Wind and Solar Power Forecasting
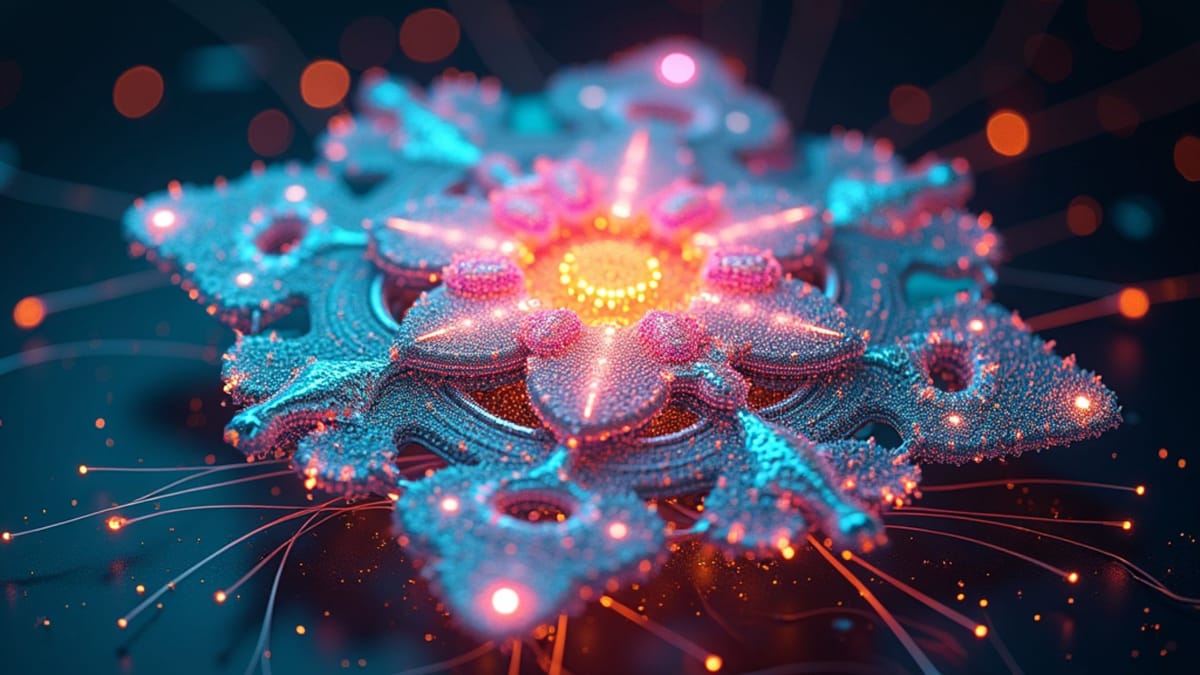
Renewable energy installations may soon benefit from hyper-specialized weather forecasts generated within minutes on standard desktop computers, eliminating the need for supercomputing resources and years-long development cycles.
End of Miles reports this breakthrough comes from Aardvark Weather, a new end-to-end AI forecasting system developed through international collaboration between the University of Cambridge, Alan Turing Institute, Microsoft Research, and the European Centre for Medium-Range Weather Forecasts (ECMWF).
Transforming Renewable Energy Planning
The revolutionary aspect of Aardvark's design lies in its ability to create application-specific weather predictions that can be tailored for particular sectors. For renewable energy operations, this means precise wind speed forecasts for turbine arrays or solar radiation predictions for photovoltaic installations without requiring the extensive computational overhead of traditional models.
"Aardvark reimagines current weather prediction methods offering the potential to make weather forecasts faster, cheaper, more flexible and more accurate than ever before, helping to transform weather prediction in both developed and developing countries." Richard Turner, researcher at the Alan Turing Institute and Cambridge University
What distinguishes this system from other AI meteorological models is its complete independence from traditional numerical weather prediction at any stage. The Cambridge-led research team has developed a system that yields high-resolution forecasts by taking observations directly from satellites, weather stations, and other sensors – bypassing the computational complexity that has historically limited specialized forecast applications.
From Years to Minutes
Current specialized forecast development for renewable energy applications typically requires substantial resources. Wind farm operators and solar facility managers often wait months or years for tailored predictive models that require supercomputers to execute, creating significant barriers to operational efficiency.
The Turing Institute model changes this paradigm completely. Specialized forecasts that previously demanded intensive development cycles can now be generated rapidly, potentially transforming how renewable energy facilities optimize their operations and integrate with power grids.
Practical Implications for Green Energy
For the renewable sector, forecast precision directly translates to economic value. Wind farm operators must predict generation capacity hours or days in advance to participate effectively in energy markets, while solar installations require accurate irradiance forecasts to optimize storage and distribution strategies.
The study published in Nature demonstrates that Aardvark's machine learning architecture can maintain competitive accuracy while dramatically reducing the barriers to specialized forecast development. This capability could prove particularly valuable for smaller renewable operations without access to enterprise-level forecasting resources.
"I think the true innovation in this model is more, 'Hey, we can remove the reliance on NWP models and go purely data-driven with AI and have it reasonably work.'" Aaron Hill, meteorology professor at the University of Oklahoma
The innovation comes at a critical juncture for renewable energy expansion. As more variable generation sources connect to power grids worldwide, enhanced predictive capabilities become essential for maintaining grid stability while maximizing clean energy utilization – potentially removing a significant obstacle to renewable energy scaling.