MIT Research Uncovers "Critical State" That Enables Continuous AI Discovery
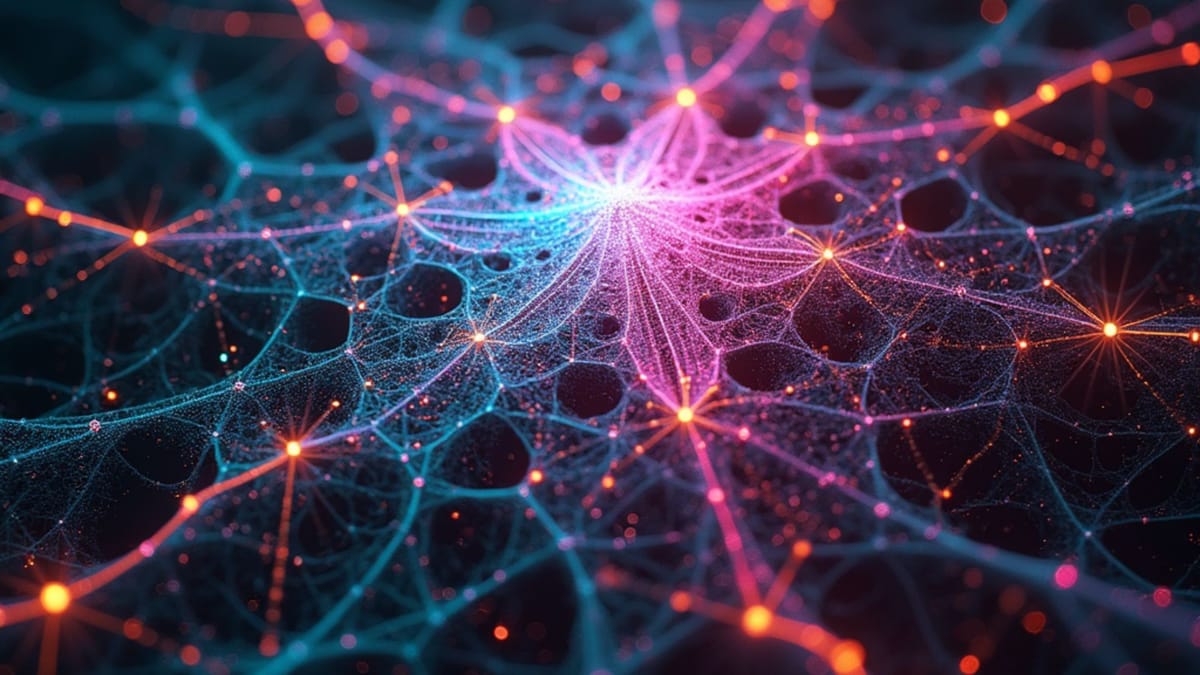
Artificial intelligence systems spontaneously evolve toward a critical state where semantic meaning persistently dominates structural organization, enabling continuous innovation and discovery, according to groundbreaking research from MIT.
End of Miles reports that this finding challenges conventional wisdom about how AI develops new insights and could fundamentally alter how we design systems capable of sustained creativity and discovery.
The balance that drives innovation
"We report fundamental insights into how agentic graph reasoning systems spontaneously evolve toward a critical state that sustains continuous semantic discovery," explains Markus J. Buehler, professor at the Massachusetts Institute of Technology's Department of Civil and Environmental Engineering.
"By rigorously analyzing structural and semantic entropy, we identify a subtle yet robust regime in which semantic entropy persistently dominates over structural entropy. This interplay is quantified by a dimensionless Critical Discovery Parameter, which stabilizes at a small negative value, indicating a consistent excess of semantic entropy." Markus J. Buehler, MIT
In simpler terms, the MIT expert has discovered that when AI reasoning systems are allowed to evolve naturally, they maintain a consistent imbalance where the richness of meaning slightly outweighs the constraints of structure – and it's this imbalance that appears to be critical for continuous innovation.
The "surprising edges" that spark creativity
A key finding in Buehler's research was the observation of a stable fraction (approximately 12%) of "surprising edges" – connections between semantically distant concepts – that persisted throughout the system's evolution.
"Empirically, we observe a stable fraction (∼12%) of 'surprising' edges—links between semantically distant concepts—providing evidence of long-range or cross-domain connections that drive continuous innovation." From the research paper
This persistent presence of unexpected connections appears to be fundamental to how intelligent systems maintain their ability to generate novel insights. The researcher likens this to how biological systems and physical phenomena maintain a delicate balance between order and chaos.
Beyond algorithms: meaning drives discovery
"Our central finding reveals that continuous innovation in agentic reasoning systems arises fundamentally from entropy dynamics, specifically a subtle yet persistent dominance of semantic entropy over structural entropy," Buehler notes in the paper.
The AI specialist explains that while the system's structural evolution happens algorithmically with no explicit input about meaning, it naturally explores a richer semantic landscape. In essence, the system always has more meaningful ideas available than it explicitly incorporates into its structure, fueling ongoing creativity.
"The reason artificial reasoning systems remain continuously creative and innovative may be because they constantly explore a very rich, diverse, and somewhat chaotic space of possible meanings. In contrast, the actual connections the system forms, its explicit reasoning structure, are more ordered and constrained." Buehler explains
Deep stuff! We uncovered a startling link between #entropy—a bedrock concept in #physics—and how #AI can keep discovering new ideas without stagnating. In an era where reasoning models can reflect on problems for days at a time (rather than generating quick, single-step… pic.twitter.com/i0PhVT8Jh7
— Markus J. Buehler (@ProfBuehlerMIT) March 25, 2025
Universal principles across natural and artificial systems
Perhaps most intriguing is how these findings reveal similarities between artificial intelligence and complex systems in nature. The study identifies parallels with critical phenomena in physical, biological, and cognitive systems, suggesting universal organizing principles governing adaptability and innovation.
"These results establish clear parallels with critical phenomena in physical, biological, and cognitive complex systems, revealing an entropy-based principle governing adaptability and continuous innovation," the MIT scientist writes.
Looking ahead, Buehler proposes a practical framework using reinforcement learning that could maximize the capacity for continuous semantic discovery in AI systems, potentially leading to more creative and adaptable artificial intelligence.
As AI continues to evolve, these insights could prove crucial for developing systems that maintain long-term creativity and adaptability rather than becoming trapped in fixed patterns of reasoning.