Mistral CEO Reveals Three-Tier AI Strategy: Specialization Is Key to Sovereign AI
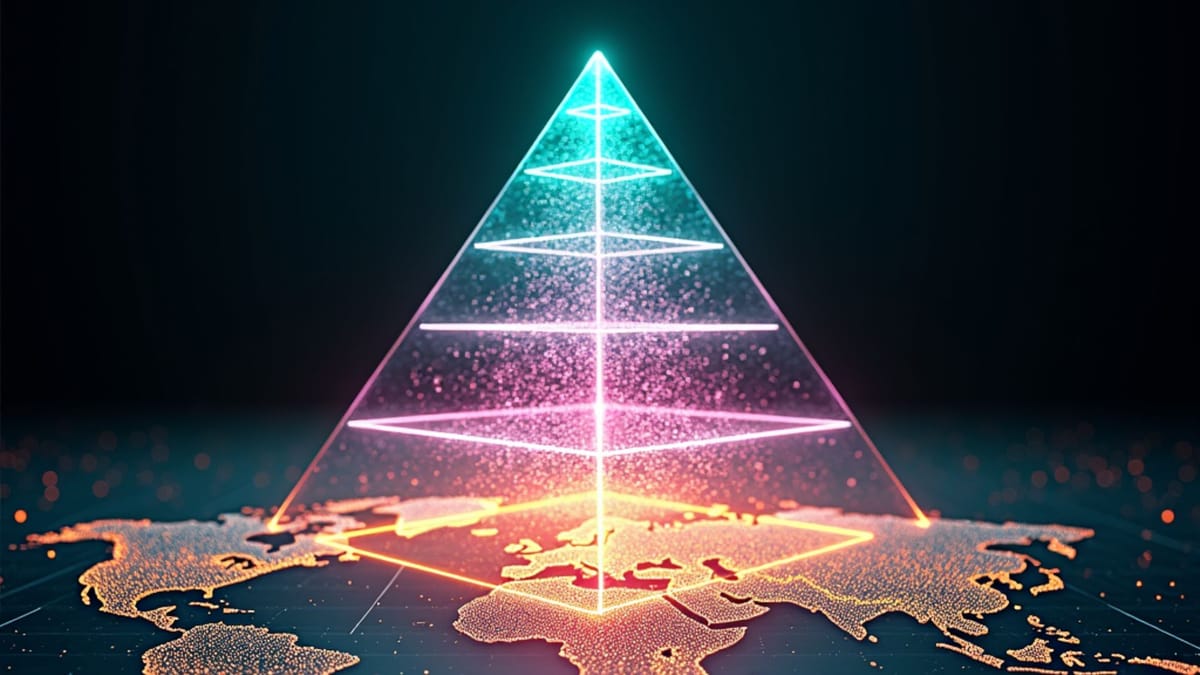
"You will have a tree of AI systems that are more and more specialized," revealed Arthur Mensch, describing how focused AI models can dramatically outperform larger, general-purpose systems despite having fewer parameters and requiring less computing power.
This three-tier approach to AI development forms the core of Mistral AI's competitive strategy against tech giants with vastly greater resources, writes End of Miles.
The Three-Layer Strategy
The Mistral CEO outlined a specific hierarchy for effective AI deployment that begins with general foundation models before adding layers of increasing specialization. Rather than pursuing ever-larger models like some competitors, Mensch's company is betting on precision.
"You will have a general purpose model and then some layer of specialization for industries and then an extra layer of specialization for companies and for countries," Mensch explained. "For a given size of model, you can get one that is much better if you choose to specialize it." Arthur Mensch, Mistral AI CEO
The AI leader didn't just theorize—he offered concrete evidence of this approach's effectiveness by highlighting Mistral's recent product development trajectory. In January, the company released Mistral Small, a general-purpose foundation model, before embarking on creating specialized variants.
Outperforming Giants Through Specialization
The French AI executive pointed to their Arabic-specialized model as proof that targeted development can overcome raw computational advantages held by larger players like OpenAI and Anthropic.
"Our model, it's the 24B, it's called M Saaba, it's a model tuned on Arabic... is outperforming every other language model that are like five times larger. And the reason for that is that we did the specialization." Mensch
This efficiency gain through specialization represents a potential breakthrough for smaller nations and organizations unable to match the massive computing resources deployed by leading AI labs. The Paris-based executive's strategy suggests that cultural and linguistic nuance can provide competitive advantages against raw scale.
Vertical Expertise Requires Partnership
The Mistral founder emphasized that specialized applications require deep collaboration between technology providers and domain experts. Using legal AI as an example, he illustrated how building effective specialized systems requires multi-layered refinement.
"If you want to build a model which is not only good at Arabic but also good at handling legal cases in Saudi Arabia, for instance, well you need to specialize it again. There's some extra work that needs to be done in partnership with companies." The Mistral CEO
This approach challenges the conventional wisdom that the largest, most general models will inevitably dominate the AI landscape. Instead, Mensch envisions a future where specialized AI systems thrive in specific domains—particularly when aligned with cultural, linguistic, and professional knowledge that global models might lack.
The Implications for National AI Strategy
For governments developing sovereign AI capabilities, the specialization strategy offers a viable path to technological independence without requiring the massive investments needed to build frontier general-purpose systems. Countries can focus resources on customizing open foundation models to their specific language, culture, and regulatory requirements.
The French tech executive's three-tier approach provides a blueprint for nations seeking AI sovereignty: adopt or develop basic foundation models, specialize them for key national industries, then further refine them for specific institutional needs.
As global competition for AI advancement intensifies, Mistral's focus on specialization over scale presents an alternative path forward—one that might prove more accessible to the majority of countries and organizations unable to match the resource expenditures of the current AI leaders.