Meta's New AI Approach Could Transform How Robots Understand Physical Reality
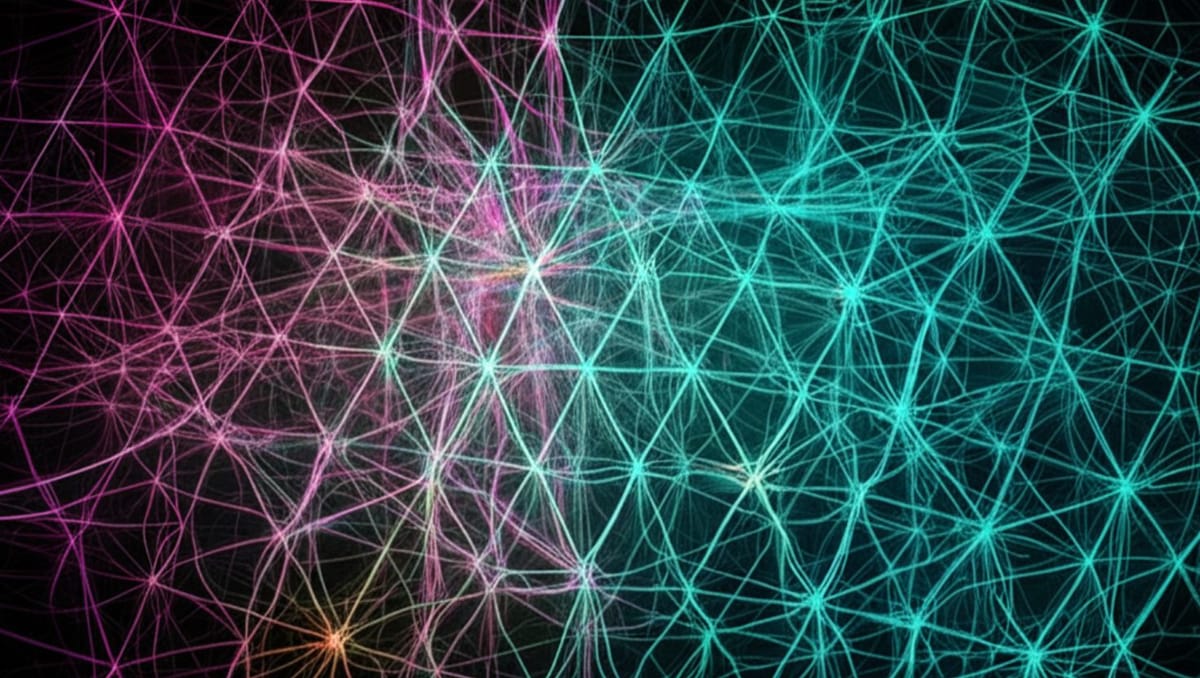
Meta's researchers have developed AI systems capable of intuitively understanding physical objects and their interactions in ways that could fundamentally transform robotics and autonomous vehicles, moving them beyond the brittle, rule-based systems that currently dominate the industry.
The breakthrough, which allows machines to develop intuitive physics understanding through a novel representation space approach, could help solve one of the most persistent problems in robotics, writes End of Miles.
Why physics understanding has been an AI blind spot
"While we've seen rapid progress in producing AI systems that often surpass human performance on high-level cognitive tasks like language, coding or mathematics, these same systems paradoxically struggle with common sense physical understanding," explains Quentin Garrido, Meta researcher and lead author of the recently published findings.
"This is Moravec's paradox in action—tasks trivial for biological organisms can be remarkably difficult for artificial systems, and vice versa." Meta AI Research
The research team demonstrated that by training neural networks to predict masked regions in natural videos using a learned representation space, they achieved remarkable results in understanding intuitive physics concepts. The Meta system achieved 98% accuracy on the IntPhys benchmark, vastly outperforming other approaches including Google's Gemini 1.5 Pro and other advanced systems.
Representation learning: The missing link for autonomous systems
What makes the approach particularly promising for autonomous vehicles and robotics is that it occupies a middle ground between competing approaches. Rather than using hand-coded rules or direct pixel prediction, the system learns to predict in an abstract representation space that captures the physics of how objects behave.
Meta AI believes this approach could significantly advance capabilities in real-world robotic systems.
"Current autonomous systems often fail in unexpected situations because they don't truly understand physical causality. Our approach suggests machines can develop this understanding through observation, similar to how infants learn about their world." Meta AI
Remarkably, the Meta researchers found that even smaller models (115 million parameters) and systems trained on just one week of video achieved significant physics understanding, suggesting the approach could be practical for deployment in resource-constrained environments like robots and vehicles.
What this means for the future of autonomous systems
The ability to understand object permanence, continuity, shape consistency, and other physics concepts without explicit programming could allow future autonomous vehicles to better predict how objects—from pedestrians to other vehicles—will behave in complex situations.
"The challenge for autonomous vehicles has never been identifying objects—it's been predicting how those objects will behave in novel situations," the Meta team noted. "Our approach shows that machines can learn to predict physical behaviors in ways that could be more robust and generalizable than current methods."
The research team is particularly optimistic about how their approach differs from current robotics systems that typically rely on simulators with hard-coded physics rules.
"Through the results reported here, we believe that the latent prediction framework is a path forward toward building neural networks that understand the physical world." Meta Research Team
For robotics applications, this intuitive understanding could translate to robots that can manipulate objects more naturally—anticipating how objects will move when pushed, pulled, or stacked, without requiring explicit programming for each scenario.
While the Meta team acknowledges that current V-JEPA models have limitations in memory and in handling interactions between objects, the promising results suggest a new direction for developing AI systems that truly understand physical reality—a critical step toward more capable and reliable autonomous vehicles and robots.