Meta's LeCun Unveils Revolutionary "JEPA" Architecture to Replace Current AI Paradigms
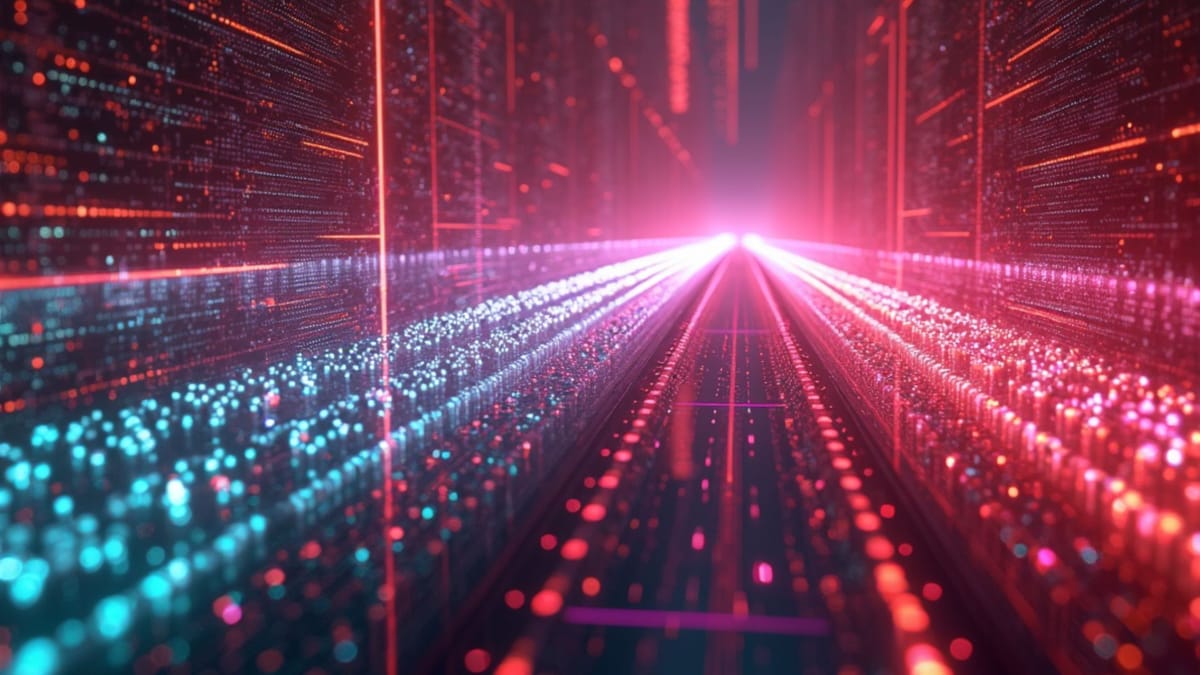
"Current generative AI approaches are fundamentally flawed and should be completely abandoned," declares Meta's Chief AI Scientist Yann LeCun, positioning a new architectural paradigm called Joint Embedding Predictive Architecture (JEPA) as the solution to what he characterizes as insurmountable limitations in today's dominant AI approaches.
The stark assessment and proposed alternative framework were presented during LeCun's prestigious Josiah Willard Gibbs lecture at the American Mathematical Society conference, End of Miles reports.
Why current approaches fail
LeCun identifies a critical mathematical limitation in current generative AI systems. These systems, when tasked with predicting high-dimensional continuous outputs like video frames, produce blurry, averaged results that fail to capture the physical world's complexity.
"If you train a system to make a single prediction, what you get are blurry predictions because the system can only predict the average of all the possible futures that may happen." Yann LeCun, Meta Chief AI Scientist
The NYU professor explains that while auto-regressive prediction works effectively for discrete symbols like text tokens, it fundamentally breaks down when applied to continuous, high-dimensional spaces where representing probability distributions becomes intractable.
The JEPA solution
The JEPA architecture represents a pivotal shift in approach. Rather than attempting direct prediction in raw input space, JEPA employs separate encoders that transform both inputs and outputs into abstract representations before prediction occurs.
The Meta researcher illustrated the key difference: in generative models, systems minimize prediction error directly between inputs and outputs, while JEPA architectures perform prediction in a representation space deliberately designed to ignore unpredictable details.
"Instead of spending a huge amount of resources attempting to predict things that you don't have enough information for, just eliminate it from the prediction process by learning representations where those details are eliminated." LeCun
This elegantly addresses a fundamental problem in world modeling—the impossibility of predicting exact pixel values for future frames when many details are inherently unpredictable from available information.
Evidence of effectiveness
The AI scientist presented evidence that JEPA models have demonstrated surprising capabilities. When tested on video data, these systems show elevated prediction errors when shown physically impossible scenarios—such as objects floating unsupported or disappearing spontaneously—despite never being explicitly trained to identify physical impossibilities.
"Those systems have kind of learned a very basic form of common sense, a little bit like the babies I was talking about earlier," the researcher noted, referencing earlier points about infant development of intuitive physics through observation.
Implications for AI's future
LeCun's recommendation to "abandon generative models in favor of joint embedding architectures" sits in notable tension with the industry's current heavy investment in generative AI, including substantial resources allocated by Meta itself.
The technical leader positioned JEPA as just one element of a comprehensive paradigm shift needed to advance toward truly intelligent systems, alongside transitions from probabilistic models to energy-based models, from contrastive to regularized methods, and from reinforcement learning to model predictive control.
For a technology leader whose company has invested billions in AI research, this public divergence from current industry trends signals a potential turning point in how researchers approach the fundamental architectures underlying artificial intelligence systems.