Meta's Chief Scientist: Industry-Standard AI Has Hit "Diminishing Returns"
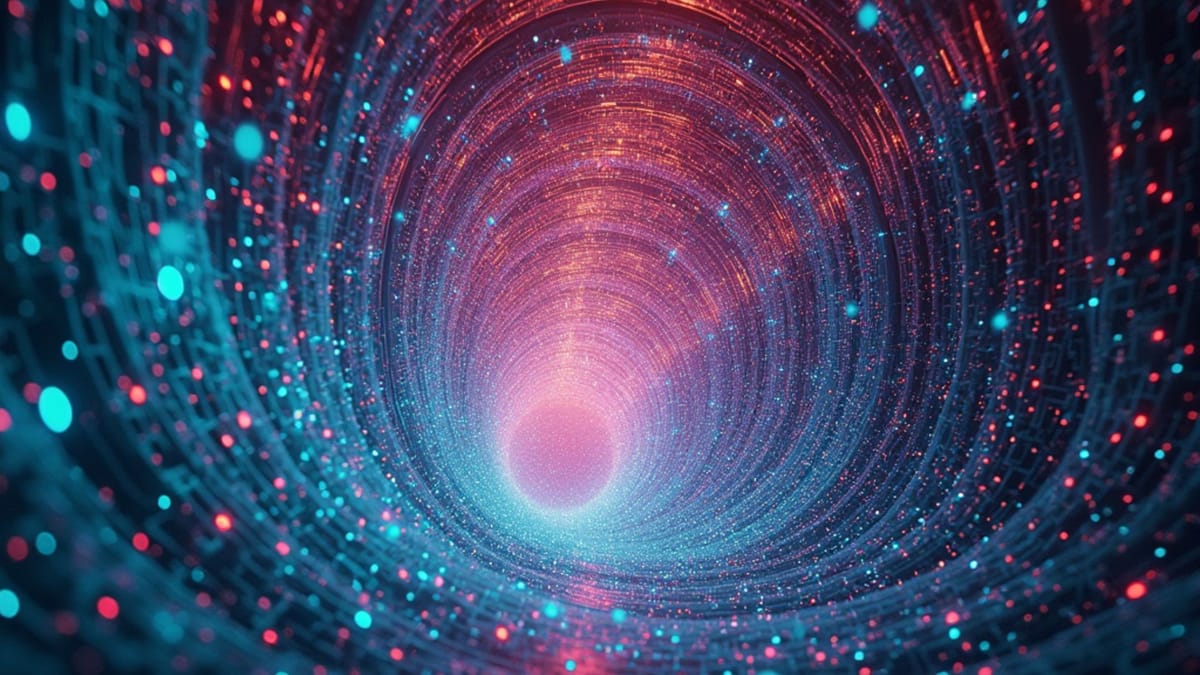
"We've kind of run out of natural text data to train those LLMs. They're already trained with you know on the order of 10 to the 13 or 10 to the 14 tokens. That's a lot. That's the whole internet," states Yann LeCun, looking directly at the technological ceiling that current AI systems are approaching.
End of Miles reports that this fundamental limitation—rarely acknowledged by companies racing to secure billion-dollar investments—represents a critical inflection point in artificial intelligence development as the field's most decorated scientists begin publicly questioning the trajectory of today's dominant models.
The mathematical dead end
The Turing Award winner's assessment cuts through industry hype with scientific precision. Current language models—the technology behind systems like ChatGPT and Claude—are approaching what LeCun describes as "diminishing returns" where massive additional investments yield increasingly modest improvements.
"The costs are ballooning of generating that data and the returns are not that great. So we need a new paradigm." Yann LeCun, Meta's Chief AI Scientist
This declaration from Meta's AI patriarch arrives as companies continue pouring unprecedented capital into scaling these same systems. OpenAI recently secured $6.6 billion in funding while Anthropic raised $7.5 billion over the past year—investments predicated largely on the assumption that simply expanding existing architectures will yield increasingly intelligent systems.
Why bigger isn't better anymore
The French-born AI pioneer identifies several concrete barriers preventing current systems from advancing toward genuine intelligence, regardless of size. As available text data becomes exhausted, companies resort to increasingly expensive and diminishingly effective alternatives.
"There is talks about generating artificial data and then hiring thousands of people to kind of generate more data, other knowledge, PhDs and professors... but it's diminishing return." LeCun
The Meta scientist's assessment contradicts the public messaging of several AI startups that have suggested their path to artificial general intelligence requires primarily additional scale rather than architectural breakthroughs.
What happens next?
LeCun's frank evaluation signals a potential strategic divide in AI development approaches. While acknowledging the usefulness of current systems, the renowned researcher emphasizes that a new architecture is required—one capable of understanding the physical world, reasoning, planning, and maintaining persistent memory.
"We are not going to get to human level AI by just scaling up LLMs. This is just not going to happen. Whatever you can hear from some of my more adventurous colleagues, it's not going to happen within the next two years. There's absolutely no way." The Turing Award recipient
This technical assessment from one of the field's founding figures raises questions about the long-term viability of business models and investment theses built primarily around scaling current architectures, potentially signaling the beginning of a recalibration in how the industry approaches the development of more capable AI systems.