Meta's Chief AI Scientist: We've Run Out of Natural Text Data for Training
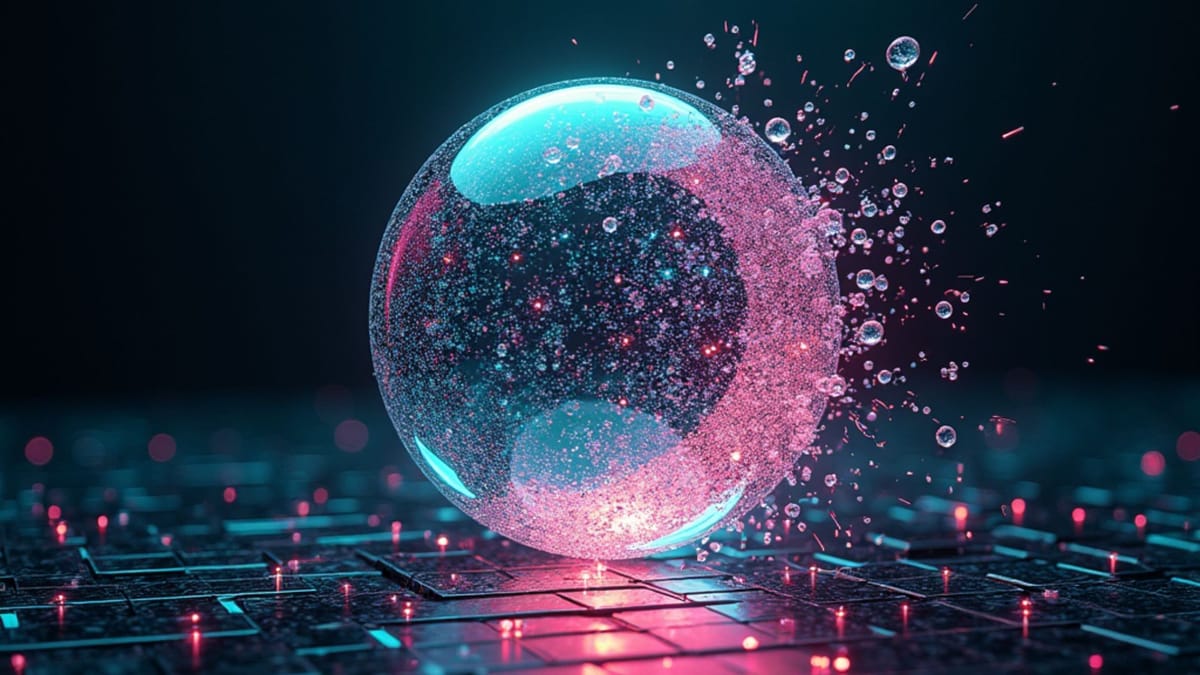
"We've basically run out of natural text data," declares Yann LeCun, setting down a stark reality check for an industry racing toward increasingly powerful AI systems. The Meta chief AI scientist's assessment cuts through the typical industry optimism about endless scaling possibilities.
This scarcity represents a fundamental constraint on current AI development approaches, writes End of Miles, with potentially far-reaching implications for the trajectory of artificial intelligence research and commercial applications.
The data ceiling no one wants to acknowledge
According to LeCun, major AI companies have already trained their models on effectively all available high-quality text on the internet. This exhaustion of what was once considered an unlimited resource now forces expensive alternatives that threaten the economics of current development approaches.
"We're already trained with you know on the order of 10 to the 13 or 10 to the 14 tokens—that's a lot, that's a lot—and that's the whole internet, that's all the publicly available internet." Yann LeCun, Meta Chief AI Scientist
The Turing Award winner explains that as natural data sources dry up, companies are resorting to increasingly expensive workarounds. These include licensing non-public content, generating synthetic data, and hiring specialized teams to create custom training materials—all with diminishing returns on investment.
Why this resource constraint matters now
This revelation comes at a critical moment when AI funding is reaching unprecedented levels, with billions flowing into companies like OpenAI ($6.6 billion) and Anthropic ($7.5 billion) on the assumption that current approaches will continue yielding proportional improvements with additional investment.
"The costs are ballooning of generating that data and the returns are not that great. So we need a new paradigm." LeCun
The AI pioneer's assessment suggests that the industry faces a pivotal inflection point: either find fundamentally new architectures for AI systems or face dramatically diminished returns on massive investments. This reality check comes as companies and investors continue to pour resources into existing approaches.
The search for alternatives
Meta's chief scientist outlined several stopgap measures companies are currently employing to address the data shortage, though he expressed skepticism about their long-term viability:
"There is talks about generating artificial data and then hiring thousands of people to generate more data, other knowledge PhDs and professors... but in fact it could be even simpler than this because most of the systems actually don't understand basic logic." LeCun
The researcher's comments suggest that these expensive workarounds might temporarily extend the current paradigm but cannot solve the fundamental limitations of today's AI architectures.
Through his technical assessment, LeCun frames the data scarcity not merely as a temporary obstacle but as evidence that AI development needs to pivot toward entirely new approaches. This perspective challenges the prevailing narrative that simply scaling existing systems with more data and computing power will inevitably lead to increasingly capable AI.
What's next for AI development
The Stanford professor advocates for new AI paradigms that don't rely exclusively on massive text datasets—specifically highlighting approaches that incorporate physical world understanding, reasoning capabilities, and planning functions. Without such a shift, he suggests the industry risks hitting a capability ceiling despite massive financial investments.
Rather than focusing solely on larger models and more training data, LeCun proposes that the future of AI requires entirely different architectures that can learn more efficiently from diverse information sources and develop more robust reasoning capabilities.
"It's diminishing returns in the sense that we've kind of run out of natural text data to train those LLMs. We're already trained with, you know, on the order of 10 to the 13 or 10 to the 14 tokens, that's a lot, that's a lot." Meta's AI chief
For an industry accustomed to solving problems through scale, this resource constraint represents a profound challenge that may force a reevaluation of dominant approaches to artificial intelligence development—and potentially reshape which companies and research groups lead the next phase of AI innovation.