Meta's AI Shows Stunning Physics Understanding After Just 128 Hours of Training
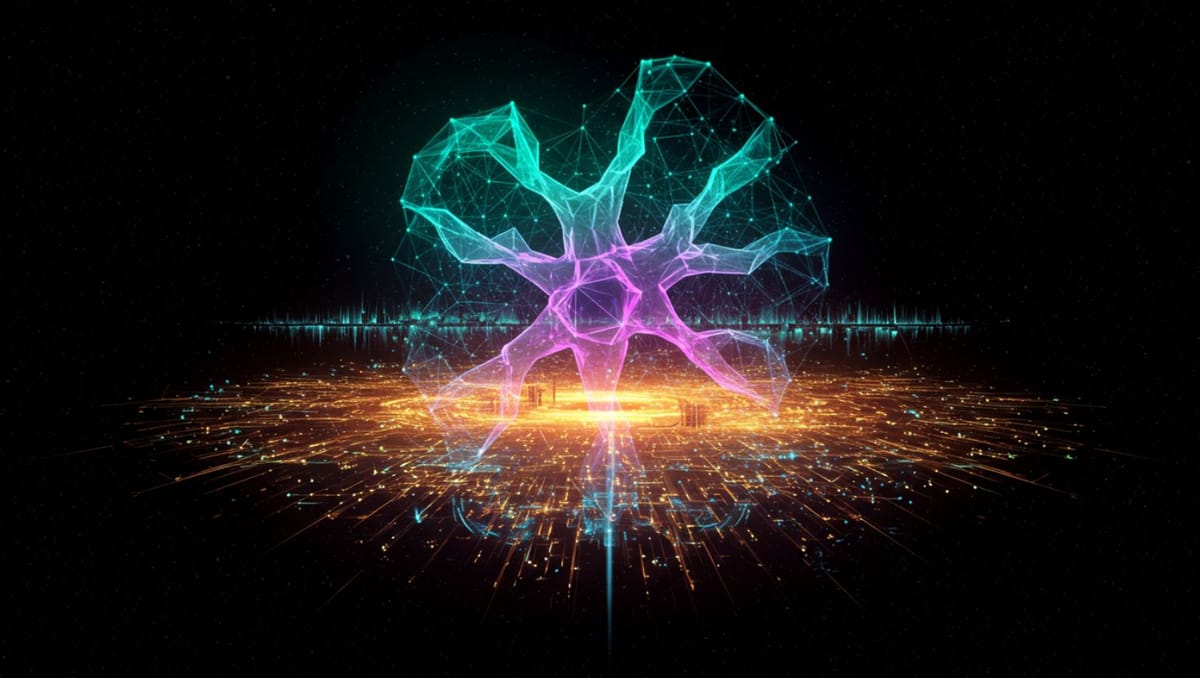
Meta's AI system developed a sophisticated understanding of intuitive physics after training on just 128 hours of video — equivalent to one week of unique visual data — dramatically challenging industry assumptions about the massive datasets required for advanced AI capabilities.
End of Miles informs that this breakthrough, detailed in Meta's February 2025 research paper, demonstrates that AI models can learn fundamental physics concepts with significantly less data than previously believed necessary, potentially transforming how future AI systems are trained and developed.
Surprising Efficiency in Learning
"We find in Figure 3.C that the size of the dataset does not meaningfully impact performance, and that the model can adequately distinguish violations of intuitive physics concepts even with 128h of unique videos, maintaining a pairwise accuracy of over 70% on all considered properties," the Meta researchers wrote in their paper titled "Intuitive physics understanding emerges from self-supervised pretraining on natural videos."
"High scores are found with only 1289 hours of Howto100M (the largest dataset), and even 128h gives better than chance performance." Meta Research Team
This finding directly challenges the prevailing assumption in AI development that mastering physics understanding requires exposure to vast amounts of training material. The research team demonstrated that their system, named V-JEPA (Video Joint Embedding Predictive Architecture), achieved surprisingly high performance even when trained on a tiny fraction of available data.
Outperforming More Complex Systems
The efficiency doesn't come at the cost of performance. Meta's system achieved up to 98% accuracy on the IntPhys benchmark for physics understanding, significantly outperforming much more complex systems including Google's Gemini 1.5 Pro and other advanced multimodal AI models that performed at near-chance levels on the same tasks.
What makes this achievement remarkable is that competing systems typically train on orders of magnitude more data. The V-JEPA approach focuses on prediction in what the researchers call "representation space" rather than the pixel-based or text-based approaches used by other systems.
"Our comparisons of these architectures reveal that jointly learning an abstract representation space while predicting missing parts of sensory input, akin to predictive coding, is sufficient to acquire an understanding of intuitive physics." Meta Research Paper
CDS founding director Yann LeCun (@ylecun) and team show that deep neural networks trained on natural videos can develop intuitive physics understanding, challenging the need for hardwired core knowledge in AI systems.https://t.co/7GCnpIYy9P
— NYU Center for Data Science (@NYUDataScience) April 9, 2025
Implications for Future AI Development
This discovery could significantly reduce computational resources required for training sophisticated AI systems. While larger datasets and models do improve performance, the research shows diminishing returns after a surprisingly small threshold.
The findings also challenge fundamental theories in cognitive science and AI development. The paper directly questions the "core knowledge" hypothesis, which suggests that humans are equipped with innate systems for understanding basic properties of the world.
The research demonstrates that even small models with 115 million parameters can achieve an accuracy of over 85% on physics understanding tasks when trained with the representation space approach, suggesting that model efficiency rather than sheer size might be the key to certain types of intelligence.
For AI developers, this could mean a shift toward more targeted, efficient training methodologies rather than the current focus on ever-larger datasets and models. This approach could potentially democratize advanced AI development by reducing the computational requirements that currently favor only the largest technology companies.