Meta's AI Chief: "A House Cat Has Better Intelligence Than Our Most Advanced AI Systems"
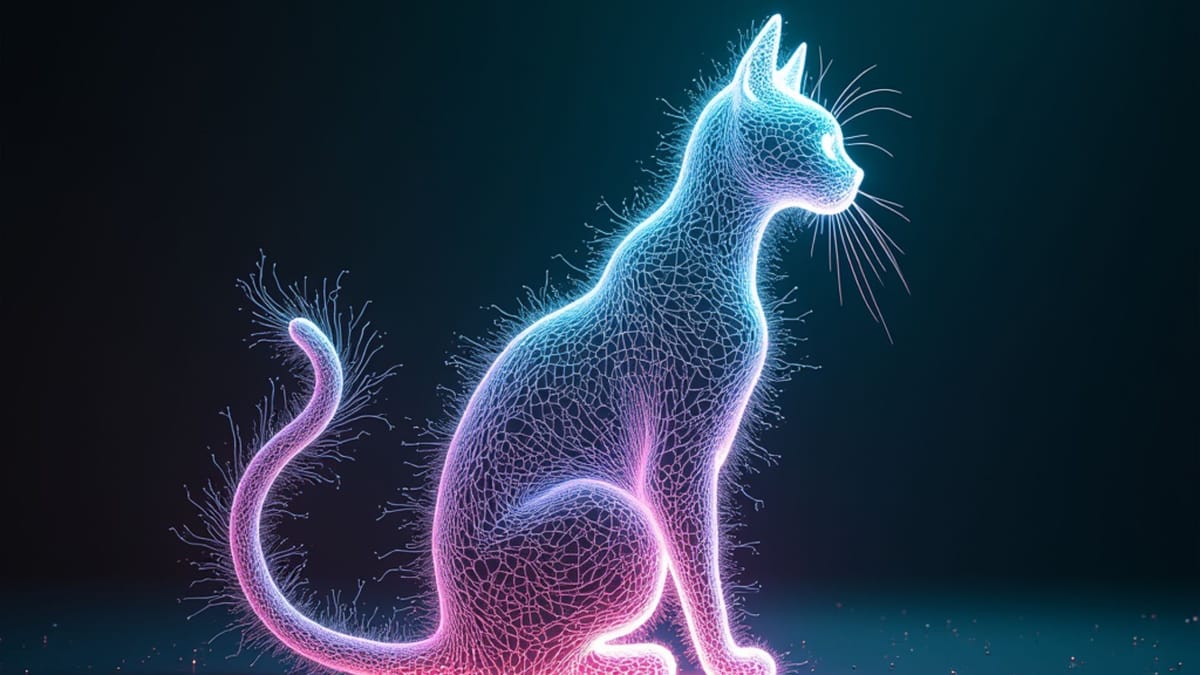
"We can't even reproduce what a cat can do," declared Yann LeCun, challenging the predominant narrative around artificial intelligence capabilities. "A cat has an amazing understanding of the physical world and I always say cat – it could be a rat – and we have no idea how to get an AI system to work as well as a cat in terms of understanding the physical world."
This striking assessment from Meta's Chief AI Scientist underscores a fundamental limitation in current AI development that remains largely overlooked amid recent advances, writes End of Miles.
The Reality Behind AI Hype
While AI systems capable of passing bar exams and solving complex mathematical problems attract headlines, LeCun focuses on a more profound deficiency: their inability to operate effectively in the physical world.
"House cats can plan really complex actions, they have causal models of the world, they know what the consequences of their actions will be," LeCun explained during his recent Josiah Willard Gibbs lecture at the American Mathematical Society. "Humans are amazing – a 10-year-old can clear up the dinner table and fill up the dishwasher without actually learning the task... because the 10-year-old has good mental models of the world." Yann LeCun, AMS Josiah Willard Gibbs Lecture
The NYU professor contrasts these innate capabilities with the massive datasets required for AI systems to perform relatively simple tasks. He points to autonomous driving as a prime example of this efficiency gap.
"A 17-year-old can learn to drive a car in 20 hours of practice, and autonomous driving companies have hundreds of thousands of training data of people driving cars around. We still don't have self-driving cars at level five." LeCun
The Moravec Paradox Revisited
This disparity exemplifies what the AI specialist identifies as the Moravec paradox – the observation that tasks difficult for humans (like playing chess or solving equations) are relatively easy for computers, while tasks that seem effortless to humans (like perception and mobility) remain extraordinarily challenging for AI.
"When people refer to human intelligence as general intelligence, that's complete nonsense," the researcher asserts. "We do not have general intelligence at all. We're extremely specialized."
The Information Asymmetry Problem
LeCun identifies a fundamental mathematical constraint limiting current AI development: the inherent information poverty of text-only training compared to multisensory learning from the physical world.
"A 4-year-old has been awake a total of 16,000 hours. We have two million optic nerve fibers, one million per eye going to the visual cortex. Each optic nerve fiber carries about one byte per second roughly. Do the calculation and that's about 10^14 bytes in four years. There's just enormously more information in sensory information that we get from vision and touch and audition than there is in all the texts ever produced by all humans." LeCun
This quantitative assessment leads to his conclusion that achieving advanced AI requires shifting away from text-dominant models toward systems that build world models from observation and interaction – much like the way infant humans and animals develop understanding.