Major AI Labs Struggle to Scale Despite Massive Cost Reductions, Reveals Tech Researcher
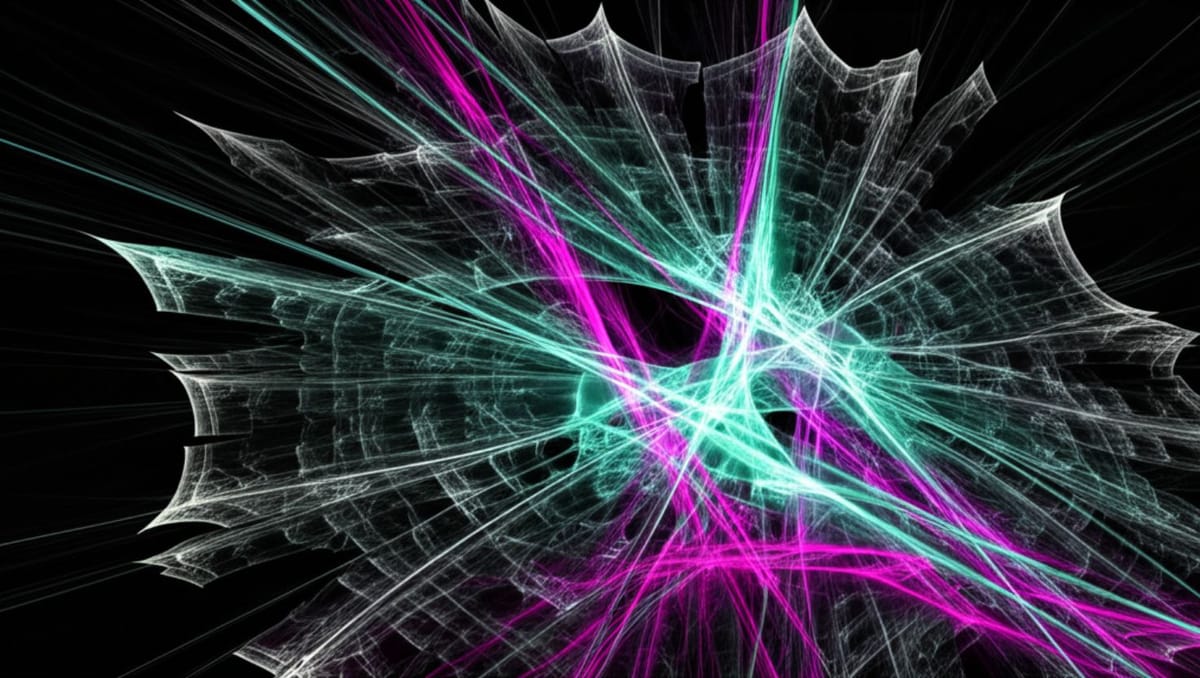
"The compute required to train a GPT-4 level model has been declining in cost at the astonishing rate of 10x per year. From the outside at least, it seems like these astonishing compute multipliers are only making existing capabilities cheaper to serve, not enabling the next generation of more powerful models to arrive much sooner," reveals leading AI researcher and podcaster Dwarkesh Patel in a candid assessment of the industry's current challenges.
End of Miles reports that this revelation contradicts the public messaging from major AI labs, which have consistently portrayed a trajectory of uninterrupted progress toward increasingly capable systems.
The unexpected scaling wall
According to Patel, rumors have been circulating that "all the labs have been struggling to crack the next OOM [order of magnitude] of scaling." This apparent plateau comes despite breakthrough after breakthrough in reducing the costs of AI training, suggesting a more fundamental challenge than mere economics.
"What's going on? Data is running out? Maybe the engineering for much larger training runs gets exponentially harder? Or maybe so-called algorithmic 'compute multipliers' don't give an equivalent multiplicative boost at different levels of scale?" Dwarkesh Patel
The tech thought leader's questions point to a significant disconnect between public messaging about AI progress and the reality inside research labs. While companies continue to tout each incremental improvement, they're apparently hitting boundaries that weren't anticipated in their scaling laws and projections.
The commodification trap
These scaling difficulties raise profound questions about the future of AI development. In his analysis, Patel considers whether foundation model companies risk commoditization if they fail to differentiate through continued capability leaps.
"Is there any moat other than staying 6+ months ahead (and in the extreme scenario beating everyone else to the intelligence explosion)? If model companies fail to differentiate, where does the value get captured?" Patel's blog
The AI researcher speculates that if progress stalls, value might shift to infrastructure providers like datacenters or even further upstream to companies producing specialized hardware components. This scenario could dramatically reshape industry power dynamics.
Implications for the AI race
This scaling barrier has significant implications for both industry strategies and national competitiveness. If current approaches are hitting fundamental limits, companies may need to explore entirely new architectures or training paradigms.
The Stanford economist's questions about how this affects investment decisions are particularly timely. "Hyperscaler AI capex is getting pretty big - approaching $100B a year for some of the big dogs. If there is a lull in AI capabilities... what will happen? Will fidgety CFOs force a firesale of compute contracts?"
"How much does being at the bleeding edge of AI capabilities matter? Is there any point in competing in the model race if you have no plan to get to the top? Or is there a viable business strategy based on being 6 months behind but following fast?" Dwarkesh Patel
The race to develop increasingly powerful AI systems has become a cornerstone of both corporate strategy and national security policy. If scaling is indeed becoming more difficult than anticipated, it could force a reevaluation of timelines for transformative AI and potentially slow the pace of disruption across industries.
What's clear from Patel's revelations is that despite the public narrative of inevitable progress, the path to more capable AI systems may be more complex and less linear than widely assumed. For an industry built on promises of exponential growth, this scaling wall represents both a technical challenge and an existential reckoning.