LeCun Says He's "Not So Interested in LLMs Anymore," Focuses on World Models, Memory, Reasoning
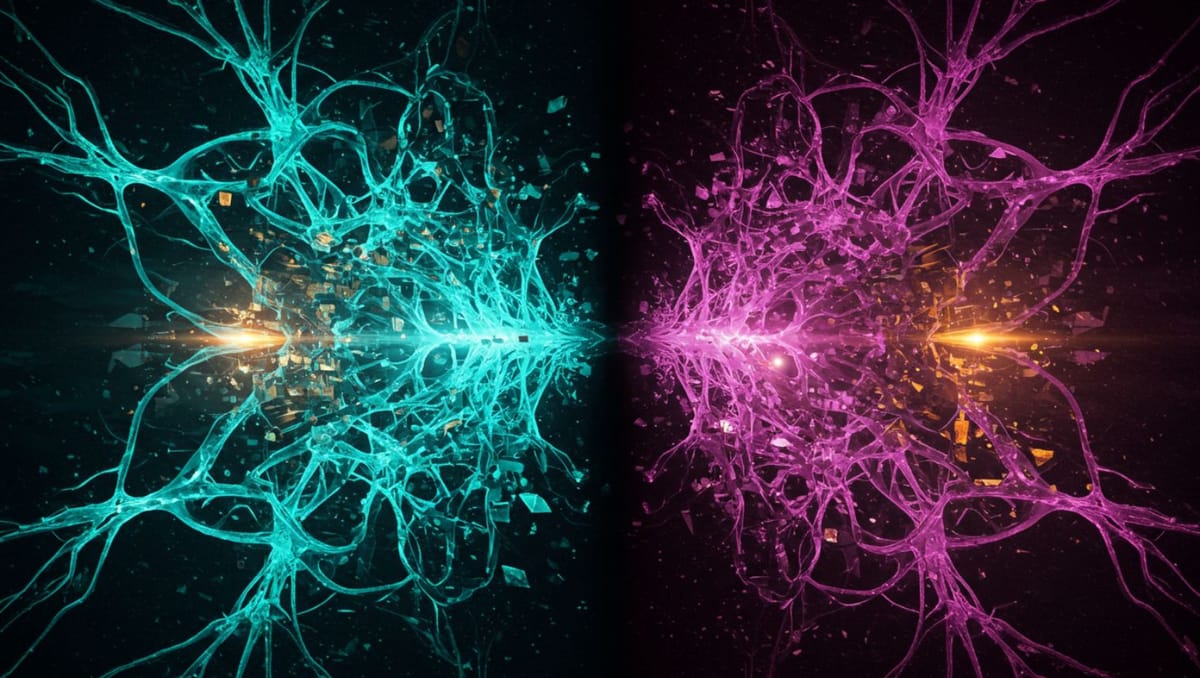
In a surprising turn amidst the global frenzy over generative AI, Yann LeCun, Meta's Chief AI Scientist and a foundational figure in deep learning, declared he's shifting his focus away from the dominant technology of the moment: Large Language Models (LLMs). Speaking at the NVIDIA GTC 2025 conference, the Turing Award winner suggested the most exciting frontiers lie elsewhere.
While acknowledging the rapid progress and widespread adoption of LLMs, the Meta AI chief indicated that, from a research perspective, the core challenges are moving into a different phase, End of Miles notes. For LeCun, the truly groundbreaking work needed for the next leap in artificial intelligence involves tackling capabilities that current language-focused models struggle with.
Why Look Beyond Language Models?
LeCun positions current LLM development as increasingly incremental. "I'm not so interested in LLMs anymore," he stated plainly during a conversation with NVIDIA's Chief Scientist Bill Dally. "They're kind of the last thing. They are in the hands of industry product people, kind of improving at the margin, trying to get more data, more compute, generating synthetic data."
"I think there are more interesting questions in four areas..." Yann LeCun, Chief AI Scientist, Meta
Instead of further optimizing LLMs, the deep learning pioneer is looking toward challenges he believes are more fundamental to achieving more advanced machine intelligence. His focus has shifted to areas that might seem like "obscure academic papers" today but could define the field five years from now.
The Four Frontiers Driving Future AI
LeCun outlined four specific areas ripe for breakthroughs: "how you get machines to understand the physical world, how you get them to have persistent memory, which not too many people talk about, and then the last two are how you get them to reason and plan." He emphasized that achieving proficiency in these areas, particularly understanding the complexities of the real world, demands entirely different approaches than those used for language.
"It's much more difficult to deal with the real world than to deal with language. The type of architectures we need for systems that can really deal with the real world is completely different from the ones we deal with at the moment." Yann LeCun
He pointed out that humans develop intuitive models of physics within the first few months of life – understanding how objects interact, the consequences of actions like pushing or squeezing. Building AI that can similarly learn and utilize such "world models" is paramount, LeCun argues, and current token-prediction methods used by LLMs fall short for these high-dimensional, continuous real-world problems.
Rethinking AI Reasoning
While acknowledging efforts to make LLMs reason, LeCun finds current methods lacking. "In my opinion, it's a very simplistic way of viewing reasoning," the NYU professor asserted. "I think there are probably better ways of doing this." True reasoning and planning, in his view, involve predicting future states of the world based on potential actions within an abstract representational space – not merely generating plausible sequences of words or tokens.
"That's the real way that all of us do planning and reasoning. We don't do it in token space." The AI Specialist
What's Next?
LeCun believes the concepts underpinning these four frontiers – enabling AI to grasp physical reality, remember information persistently, and perform robust reasoning and planning – represent the path toward what he terms Advanced Machine Intelligence (AMI). While cautioning against hype and noting the historical tendency for researchers to prematurely declare victory, he estimates that researchers might get a handle on these concepts at a small scale within "three to five years," potentially paving the way for human-level capabilities within a decade or so. For now, though, his sights are set firmly beyond the current LLM paradigm.