Language Models Could Predict How Cells Will React to Cancer Treatments
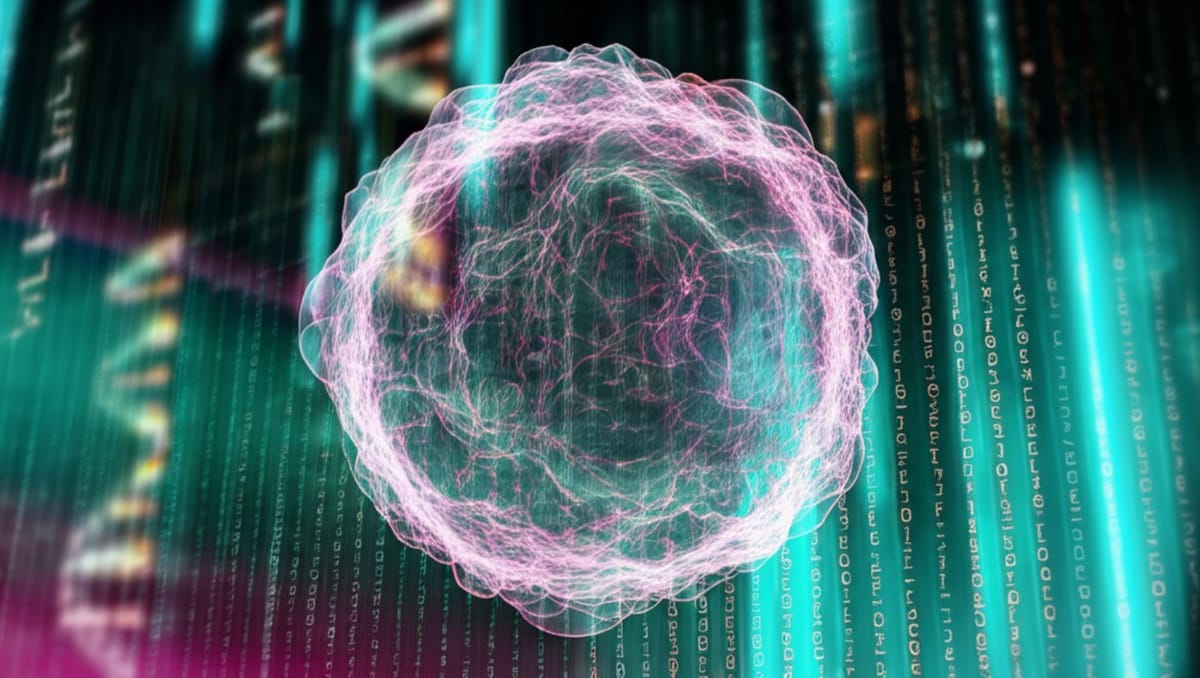
"We can now ask how a specific T cell will respond to anti-PD-1 therapy, and our AI model can answer in natural language, drawing from both cellular data and biological knowledge," explains David van Dijk, Assistant Professor at Yale University and co-developer of a groundbreaking new artificial intelligence system that could revolutionize cancer treatment planning.
End of Miles reports that researchers from Yale University and Google Research have developed AI models capable of forecasting how individual cells might respond to cancer therapies before they're administered to patients, potentially transforming how oncologists approach treatment selection.
The language of cells
The new technology, called Cell2Sentence-Scale (C2S-Scale), translates complex gene expression data from individual cells into text that large language models can interpret and analyze. This approach enables AI systems to predict cellular behavior in ways that were previously impossible outside of laboratory testing.
"One of the most exciting applications of C2S-Scale is forecasting how a cell will respond to a perturbation — like a drug, a gene knockout, or exposure to a cytokine. Given a baseline cell sentence and a description of the treatment, the model can generate a new sentence representing the expected gene expression changes." Yale and Google Research team
The Google researcher involved in the project, Bryan Perozzi, emphasized that this ability to simulate cellular behavior "in silico" could dramatically accelerate drug discovery and personalized medicine. Rather than relying solely on laboratory experiments, which can be time-consuming and expensive, oncologists might one day use these models to virtually test how different cancer treatments would affect a patient's specific cellular profile.
Transforming cancer care
For cancer patients, the implications are profound. Current approaches to cancer treatment often involve trial and error, with oncologists selecting therapies based on statistical likelihoods of success rather than predictions tailored to an individual's cellular makeup.
"This represents a major step towards creating realistic 'virtual cells', which have been proposed as the next generation of model systems — potentially offering faster, cheaper, and more ethical alternatives to traditional cell lines and animal models." Van Dijk and Perozzi
The technology shows particular promise for immunotherapies like anti-PD-1, which works by helping the immune system's T cells recognize and attack cancer. These treatments are remarkably effective for some patients but completely ineffective for others, with few reliable methods to predict response before treatment begins.
From research to clinical practice
While the Yale professor cautions that clinical application remains years away, the technology is already available for research purposes. "Cell2Sentence models and resources are now available on platforms such as HuggingFace and GitHub," the team announced in their research blog.
The research team, which spans multiple institutions including Google DeepMind, has released a family of models ranging from 410 million to 27 billion parameters. This range allows researchers with different computational resources to experiment with the technology.
"We invite you to explore these tools, experiment with your own single-cell data, and see how far we can go when we teach machines to understand the language of life — one cell at a time." Research team
For cancer researchers and physicians, the ability to predict cellular responses to specific drugs before administering them represents a significant advancement toward the long-sought goal of truly personalized medicine. As these models continue to improve following demonstrated scaling laws, their accuracy in predicting treatment outcomes could fundamentally change how cancer therapies are selected and administered.