Hassabis Proposes Revolutionary Conjecture: Classical AI May Render Quantum Computers Unnecessary for Some Physics Problems
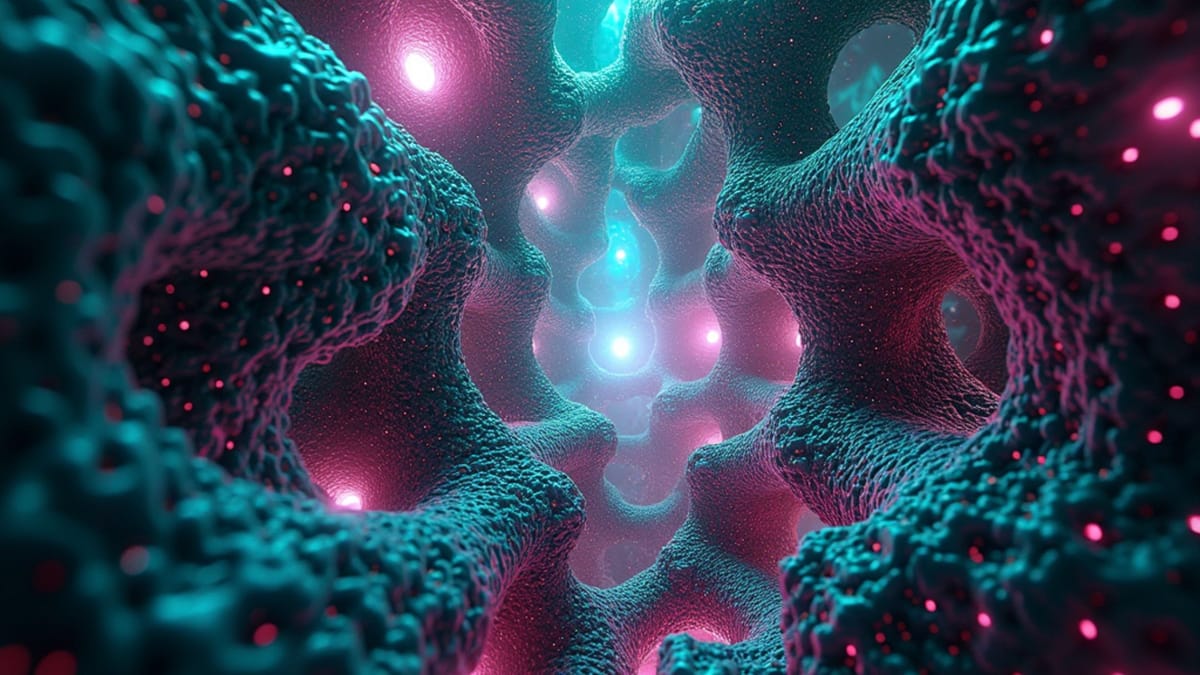
"Any pattern that can be generated or found in nature can be efficiently discovered and modeled by classical learning algorithms like AlphaFold," declared Nobel laureate Demis Hassabis during his recent Cambridge lecture, challenging fundamental assumptions about the computational requirements for modeling quantum systems.
End of Miles reports that this provocative conjecture from DeepMind's CEO represents a significant departure from conventional thinking about the limits of non-quantum computing architectures.
Rewriting computational boundaries
The Cambridge alumnus articulated a radical proposition: classical Turing machines may possess far greater capabilities than previously recognized, particularly in modeling quantum phenomena that many believed required quantum computers.
"If you think about AlphaFold and protein folding, proteins are quantum systems. They operate at the atomic scale, and one might think you need quantum simulations to actually be able to find the structures of proteins. And yet we were able to approximate those solutions with our neural networks." Demis Hassabis, Nobel Chemistry Prize recipient
This insight forms the basis of what the DeepMind co-founder frames as a fundamental conjecture about computational tractability. The implications extend beyond protein structure prediction into the domains of quantum mechanics and fundamental physics.
The AlphaFold proof point
Hassabis points to AlphaFold's success as empirical evidence for his theory. The AI system accurately predicts the three-dimensional structures of proteins—complex molecules whose behavior is governed by quantum interactions—using only classical computing architectures.
"Classical touring machines, basically classical machines that these types of AI systems are built on, can do a lot more than we previously gave them credit for." The Nobel Prize winner
The AI researcher connects this observation to the long-standing "P versus NP problem" in computer science, which explores which problems are tractably solvable on classical systems. His conjecture suggests that the boundary between quantum and classical computational domains may be significantly different than currently understood.
Philosophical implications
The implications of Hassabis's conjecture extend beyond practical computing applications. If patterns generated by quantum processes can be efficiently modeled by classical algorithms, this raises profound questions about the nature of reality itself.
"If that turns out to be true, I think that has all sorts of implications for quantum mechanics and actually fundamental physics, which is something that I hope to explore and many of my colleagues hope to explore." The DeepMind CEO
The Cambridge-educated scientist suggested that classical learning algorithms may be capable of modeling any system with physical structure that persists over time, regardless of whether that system operates according to quantum principles.
For Hassabis, this represents a return to his original motivation for pursuing artificial intelligence: creating "the ultimate general purpose tool to understand the universe around us and our place in it."