Google DeepMind Researchers Call for AI Systems to Adopt 'Lifelong Learning'
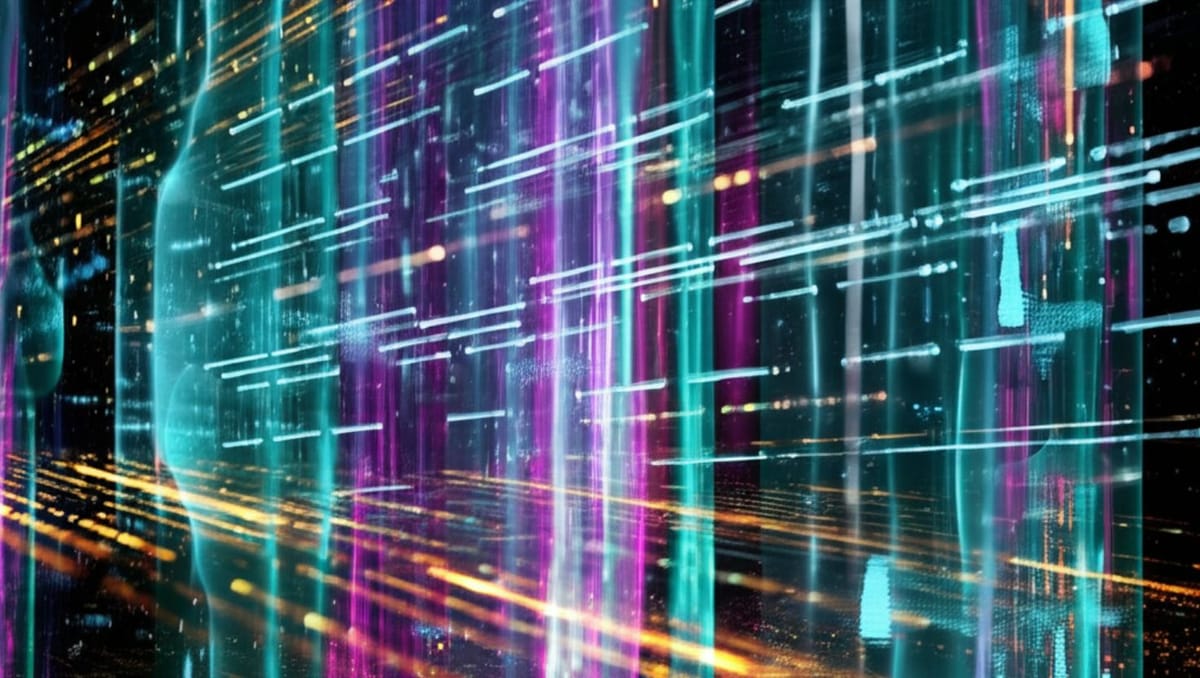
AI systems are fundamentally limited by their inability to learn continuously over time, according to two legendary researchers at Google DeepMind who are advocating for a radical shift in how artificial intelligence is developed and deployed.
In a groundbreaking paper titled "Welcome to the Era of Experience," End of Miles reports that DeepMind's David Silver and Richard Sutton argue current AI models suffer from a critical flaw: they operate in "short snippets of interaction" rather than developing through continuous experience.
The problem with current AI interactions
Silver and Sutton, both pioneers in reinforcement learning, point to a fundamental limitation in today's large language models: they're designed to answer individual queries without retaining or building upon previous interactions.
"In the era of human data, language-based AI has largely focused on short interaction episodes: e.g., a user asks a question and the agent responds," the researchers write. "Typically, little or no information carries over from one episode to the next, precluding any adaptation over time." Google DeepMind paper
This approach creates a critical bottleneck in AI development, according to the DeepMind scientists. Without the ability to learn continuously from interactions, AI remains limited to performing discrete tasks rather than developing deeper capabilities that emerge from sustained learning.
The 'streams' alternative
The AI specialists propose a new framework they call "streams" — continuous flows of experience that allow AI systems to accumulate knowledge over extended periods, similar to how humans learn throughout their lives.
"Powerful agents should have their own stream of experience that progresses, like humans, over a long time-scale,"Silver and Sutton
This represents a significant departure from the current prompt-based interaction model that dominates AI systems like ChatGPT and Google's own Gemini. The Stanford-trained researchers argue that AI systems need continuous exposure to real-world interactions to develop truly advanced capabilities.
From theoretical concept to practical applications
What makes this proposal particularly noteworthy is that Silver and Sutton believe implementing streams is possible with "today's technology." They point to recent developments like web-browsing AI agents as early steps toward more autonomous systems capable of continuous learning.
The reinforcement learning experts envision AI assistants that could track a person's health metrics over months or years, educational agents that follow students throughout their academic careers, or scientific agents that pursue long-term research goals.
"A science agent could pursue ambitious goals, such as discovering a new material or reducing carbon dioxide. Such an agent could analyse real-world observations over an extended period, developing and running simulations, and suggesting real-world experiments or interventions." From the DeepMind paper
Why this matters now
The timing of this proposal is significant. It comes as the AI field grapples with apparent plateaus in the capabilities of current large language models, despite increasing their size and training data. The Google researchers suggest that simply scaling up existing approaches won't be enough.
Silver, who led the groundbreaking AlphaZero project that mastered Chess and Go, and Sutton, a Turing Award winner for his work on reinforcement learning, carry significant weight in the field. Their call for AI systems to adopt lifelong learning principles signals a potential inflection point in how the next generation of AI systems might be developed.
The proposal also raises important questions about AI oversight, as the researchers acknowledge that systems operating over extended timeframes would provide fewer opportunities for humans to "intervene and mediate the agent's actions" — a challenge that will likely become increasingly relevant as these technologies develop.