Google and Yale Create 'Virtual Cells' That Could Replace Lab Experiments
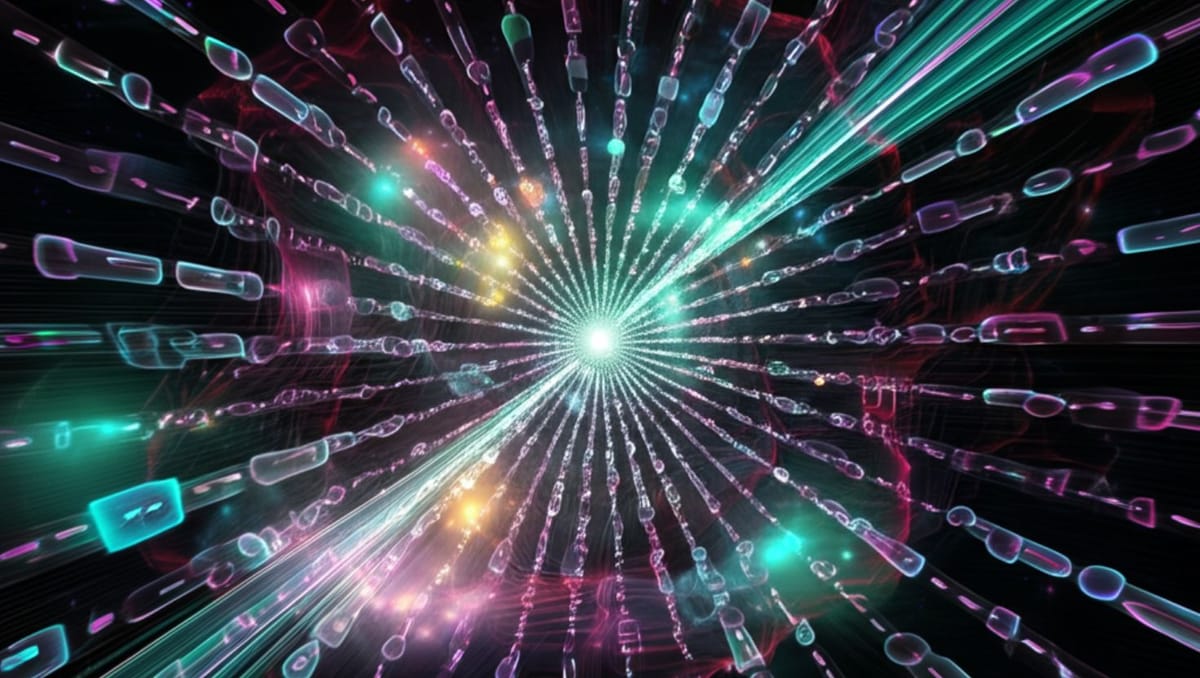
"One of the most exciting applications is forecasting how a cell will respond to a perturbation — like a drug, a gene knockout, or exposure to a cytokine," reveals Yale Assistant Professor David van Dijk about a breakthrough AI system that can simulate cellular behavior without traditional lab experiments.
End of Miles reports that this technology, called Cell2Sentence-Scale (C2S-Scale), could fundamentally transform how researchers develop and test new drugs by creating "virtual cells" that serve as faster, cheaper, and potentially more ethical alternatives to traditional lab testing.
Teaching AI to read cells like language
The Yale-Google collaboration has developed a family of large language models that can "read" and "write" biological data at the single-cell level by transforming complex gene expression profiles into simple text sequences.
"What if we could turn those thousands of numbers into language that humans and language models can understand? That is, what if we could ask a cell how it's feeling, what it's doing, or how it might respond to a drug or disease — and get an answer back in plain English?" David van Dijk and Bryan Perozzi
This approach makes highly complex biological data accessible through the same interfaces used for everyday language tools. The Yale professor's team converts each cell's gene expression into a "cell sentence" — essentially a list of the most active genes ordered by their expression level — allowing language models to process this information.
Virtual experiments before real ones
The most revolutionary aspect of this technology is its potential to predict cellular responses without physical experiments. Given information about a baseline cell and a proposed treatment, the AI can generate a new prediction representing the expected changes in gene expression.
"This ability to simulate cellular behavior in silico accelerates drug discovery, personalized medicine, and prioritizing experiments before they're performed in the lab." The research team
Google research scientist Bryan Perozzi explains that C2S-Scale represents a major step toward creating realistic "virtual cells" that have been proposed as next-generation model systems. These virtual models could potentially offer faster results with lower costs and fewer ethical concerns compared to traditional cell lines and animal testing.
Why this matters for medicine
The implications for drug development are substantial. Currently, pharmaceutical companies must conduct thousands of physical experiments to test how compounds might affect different cells. By running initial screenings virtually, researchers could identify promising candidates much earlier.
The team has already demonstrated the system's ability to predict responses to cancer therapies like anti-PD-1 treatments. "Imagine someone asking, 'How will this T cell respond to anti-PD-1 therapy?'" the Yale biologist notes. "C2S-Scale models can answer in natural language, drawing from both the cellular data and biological knowledge."
The researchers have made their models open-source, with options ranging from 410 million to 27 billion parameters to accommodate different research needs and computational resources. Performance improves predictably as model size increases, suggesting even greater capabilities may emerge with future scaling.
"We invite you to explore these tools, experiment with your own single-cell data, and see how far we can go when we teach machines to understand the language of life — one cell at a time." The research team