DeepMind Chiefs Declare End of LLM Dominance: "Era of Experience" to Replace Human Data
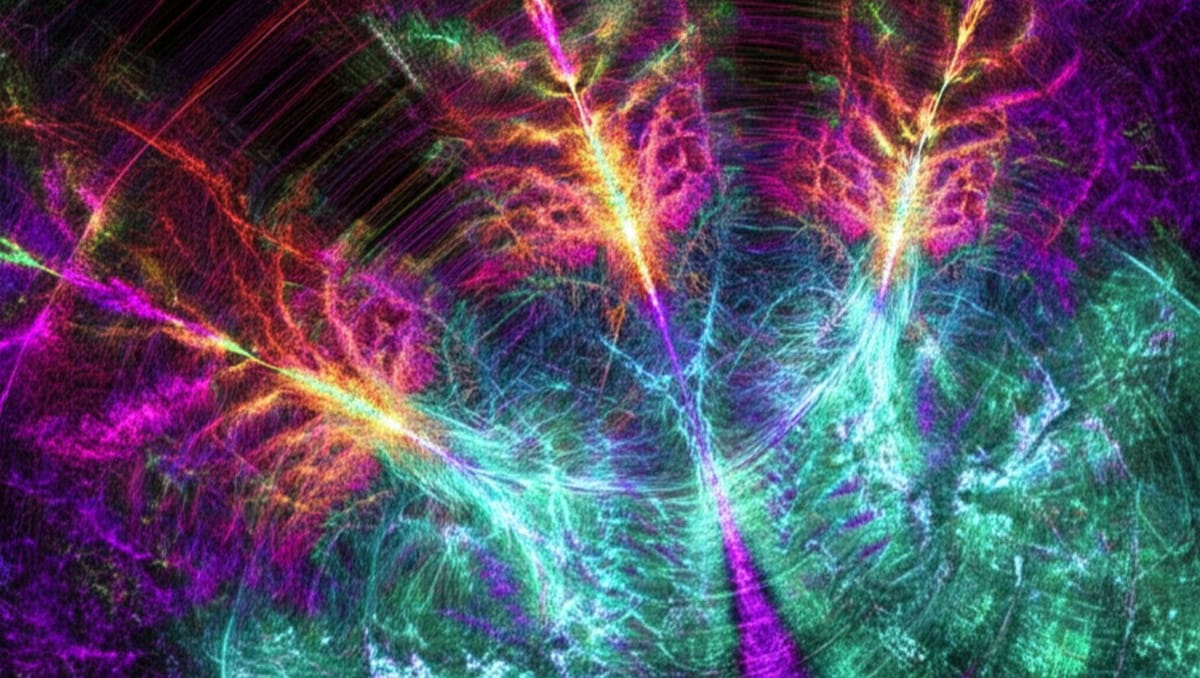
"We stand on the threshold of a new era in artificial intelligence that promises to achieve an unprecedented level of ability," declare David Silver and Richard Sutton in their groundbreaking paper that effectively announces the end of large language model dominance in AI research.
End of Miles reports that the paper, titled "Welcome to the Era of Experience", represents a significant challenge to the prevailing AI development paradigm that has driven remarkable progress in recent years.
The Limits of Human Data
The DeepMind chief scientists argue that AI systems trained primarily on human-generated data are rapidly approaching a ceiling in their capabilities, particularly in crucial domains like mathematics, coding, and science.
"While imitating humans is enough to reproduce many human capabilities to a competent level, this approach in isolation has not and likely cannot achieve superhuman intelligence across many important topics and tasks." David Silver and Richard Sutton
According to the researchers, we've nearly exhausted the utility of high-quality human data. "The majority of high-quality data sources—those that can actually improve a strong agent's performance—have either already been, or soon will be consumed," they write. "The pace of progress driven solely by supervised learning from human data is demonstrably slowing."
The Coming Era of Experience
The solution, the AI pioneers contend, is a fundamental shift toward agents that learn predominantly from their own experiences rather than human examples. This approach would enable AI to transcend the boundaries of existing human knowledge.
"To progress significantly further, a new source of data is required. This data must be generated in a way that continually improves as the agent becomes stronger... This can be achieved by allowing agents to learn continually from their own experience." From "Welcome to the Era of Experience"
The paper suggests this transition may have already begun, pointing to DeepMind's AlphaProof system, which recently became the first program to achieve a medal in the International Mathematical Olympiad. The Stanford experts explain that while AlphaProof was initially exposed to human-created formal proofs, it generated a hundred million more through continual interaction with a proving system.
Beyond Human Ways of Thinking
Perhaps most provocatively, Silver and Sutton suggest that the era of experience will produce AI systems that reason in fundamentally non-human ways.
"It is highly unlikely that human language provides the optimal instance of a universal computer," the AI luminaries write. "More efficient mechanisms of thought surely exist, using non-human languages that may for example utilise symbolic, distributed, continuous, or differentiable computations."
The computer scientists maintain that human-derived reasoning methods inherit flawed assumptions and biases from human data. To overcome these limitations, they argue AI systems must be grounded in real-world data and experience.
"Without this grounding, an agent, no matter how sophisticated, will become an echo chamber of existing human knowledge. To move beyond this, agents must actively engage with the world." Silver and Sutton
This shift represents a return to reinforcement learning principles that were somewhat sidelined during the rise of large language models. The researchers conclude that while LLMs enabled unprecedented breadth of behaviors, they also imposed a ceiling: "agents cannot go beyond existing human knowledge."