Claude 3.5 Sonnet Outperforms OpenAI Models in Complex AI Research Replication Benchmark
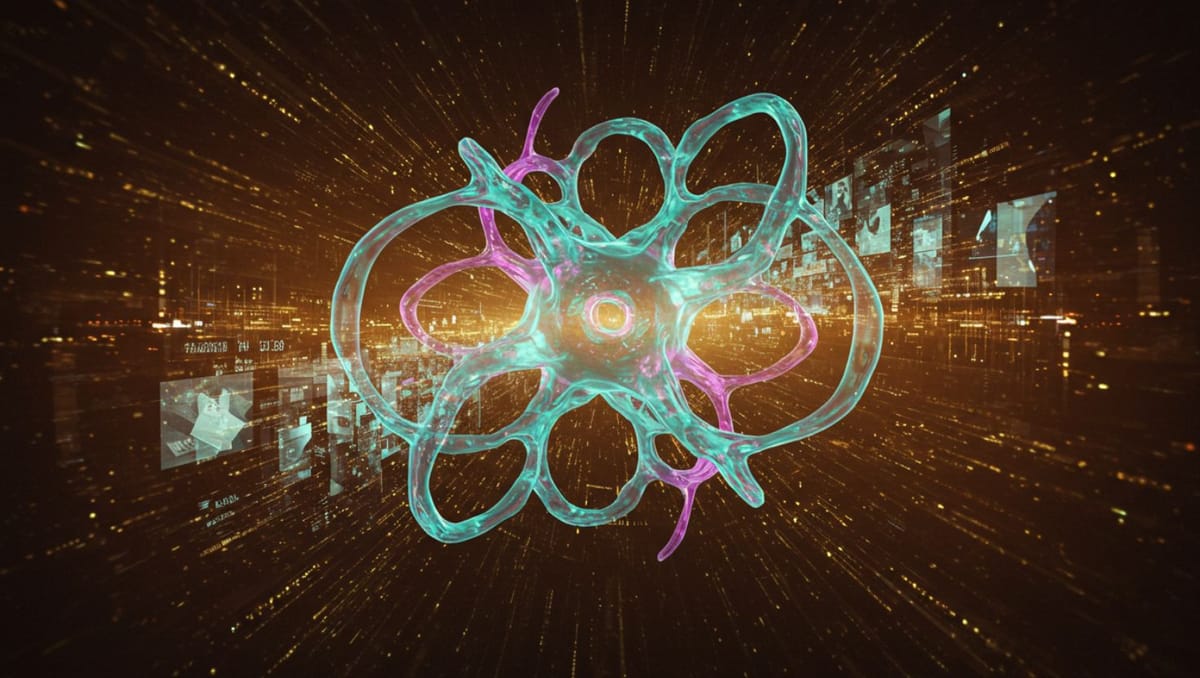
Anthropic's Claude 3.5 Sonnet has demonstrated significantly better performance than OpenAI's models in replicating complex machine learning research, achieving a 21.0% replication score compared to OpenAI's o1 model at 13.2% on a new benchmark designed to test AI research capabilities.
End of Miles reports that this performance gap suggests Claude may have distinct advantages in complex reasoning tasks involving multi-step research procedures and code implementation.
PaperBench: A New Standard for Measuring AI Research Abilities
The findings come from PaperBench, a comprehensive new benchmark developed by researchers at OpenAI that evaluates the ability of AI systems to replicate state-of-the-art machine learning research papers from scratch. The benchmark requires AI agents to understand papers, develop codebases, and successfully execute experiments without access to the original authors' code.
"Complete replication involves understanding the paper, developing a codebase from scratch to implement all experiments, and running, monitoring, and troubleshooting these experiments as needed," notes the study, which selected 20 Spotlight and Oral papers from the 2024 International Conference on Machine Learning (ICML) spanning 12 different topics.
"In general, each replication task is highly challenging and takes human experts several days of work at a minimum." PaperBench study authors
Claude's Decisive Performance Lead
When tested on PaperBench with a simple agentic scaffold, Claude 3.5 Sonnet achieved an average replication score of 21.0%, while OpenAI's o1 scored 13.2%. Other models tested performed significantly worse, with scores under 10%, including GPT-4o (4.1%), DeepSeek-R1 (6.0%), o3-mini (2.6%), and Gemini 2.0 Flash (3.2%).
The AI systems were evaluated using complex rubrics co-developed with the original authors of each research paper, resulting in 8,316 individually gradable outcomes across the 20 papers. Each paper's replication tasks were hierarchically decomposed into increasingly fine-grained requirements with clear grading criteria.
Understanding the Performance Gap
What makes Claude's performance particularly notable is that it occurred despite using the same basic scaffolding as other models. The researchers manually inspected agent logs to understand performance differences and found that most models except Claude frequently finished early, claiming they had either completed the replication or faced an insurmountable problem.
The study notes: "All agents failed to strategize about how best to replicate the paper given the limited time available to them," suggesting that even the best-performing models still struggle with time management and task prioritization in complex research settings.
When the researchers developed an alternative "IterativeAgent" scaffold that prevents early task termination, OpenAI's o1 performance improved dramatically to 24.4%, while Claude's performance with this scaffold actually decreased to 16.1%. This suggests that Claude may have inherent advantages with simpler scaffolding, while OpenAI's models benefit more from structural guidance.
Implications for AI Research Capabilities
The study provides concrete data relevant to frameworks monitoring progress toward autonomous AI research capabilities, including OpenAI's Preparedness Framework, Anthropic's Responsible Scaling Policy, and Google DeepMind's Frontier Safety Framework.
Despite Claude's relative advantage over other models, the results demonstrate that current AI systems still fall significantly short of human capabilities in complex research tasks. In a separate evaluation comparing AI with ML PhDs on a subset of papers, researchers found that humans achieved 41.4% after 48 hours of effort, compared to 26.6% achieved by o1 on the same subset.
"We observe that o1 initially outperforms the human baseline during the early stages of the replication attempt, but humans start outperforming the AI agent after 24 hours." PaperBench study authors
This pattern suggests that current AI systems excel at rapid code generation but struggle with the strategic thinking and iterative refinement necessary for complex research tasks—capabilities where Claude appears to have a modest but meaningful edge over its competitors.