Anthropic's 'Think' Tool Bridges Gap Between General AI and Domain Specialists
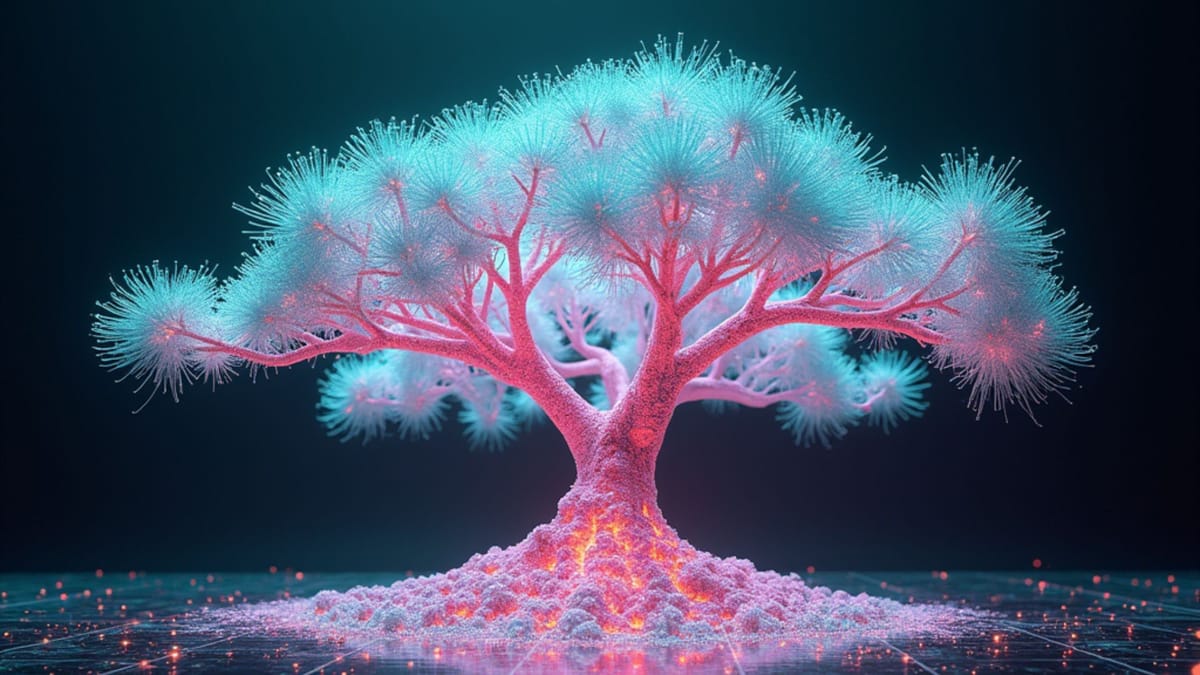
A deceptively simple new AI technique from Anthropic creates a designated "thinking space" that transforms general language models into domain specialists without extensive retraining, potentially revolutionizing how businesses implement AI across specialized industries.
End of Miles reports the new approach, detailed in Anthropic's March 20th engineering blog post, provides a critical bridge between all-purpose AI systems and the specialized capabilities enterprises need for complex industry-specific tasks.
The missing link for complex tasks
Anthropic's new "think" tool addresses a fundamental challenge in AI deployment: how to make general-purpose language models excel at complex domain-specific tasks without the cost and complexity of complete retraining. The AI lab's research reveals significant performance improvements across multiple benchmarks, particularly for tasks requiring policy adherence and sequential decision-making.
"With the 'think' tool, we're giving Claude the ability to include an additional thinking step—complete with its own designated space—as part of getting to its final answer," Anthropic explains in its engineering blog
The AI company clarifies this approach differs from their recently announced "extended thinking" capability. While extended thinking occurs before response generation, the "think" tool creates a dedicated reasoning space during the response process, particularly useful when dealing with complex tool calls or multi-step conversations.
Dramatic performance gains through simplicity
What makes Anthropic's approach particularly notable is its simplicity. Unlike other AI advancements requiring complex architectural changes, the "think" tool requires minimal code implementation while delivering outsized performance improvements.
"The 'think' tool with an optimized prompt achieved 0.570 on the pass^1 metric, compared to just 0.370 for the baseline—a 54% relative improvement." Anthropic Research Team
These improvements were most dramatic in the airline domain of Anthropic's τ-bench benchmark, with the retail domain also showing gains even without specialized prompting. This differential suggests some industries may require more customized implementation approaches than others.
The customization blueprint for enterprises
Perhaps most significantly, Anthropic's research outlines a blueprint for enterprises to transform general language models into specialized domain experts through strategic prompting with industry-specific examples. This approach could radically alter the AI implementation landscape by allowing businesses to achieve specialized performance without building custom models.
"The most effective approach is to provide clear instructions on when and how to use the 'think' tool... Providing examples tailored to your specific use case significantly improves how effectively the model uses the 'think' tool." Anthropic Implementation Guide
Anthropic recommends organizations include examples that demonstrate the level of detail expected in reasoning, how to break down complex instructions, decision trees for common scenarios, and verification steps for information collection.
Where this matters most
The AI research company identifies specific scenarios where their approach delivers the greatest value: tool output analysis requiring careful processing before action, policy-heavy environments with detailed guidelines, and sequential decision making where mistakes are costly.
Notably, the technique shows minimal value for simpler use cases like non-sequential tool calls or basic instruction following, suggesting organizations should prioritize implementation in their most complex AI workflows.
While Anthropic's research was conducted with their Claude 3.7 Sonnet model, they note that "experiments show Claude 3.5 Sonnet is also able to achieve performance gains with the same configuration," indicating the approach generalizes across models rather than being version-specific.