AI Testing Methods Fundamentally Flawed, Researcher Warns
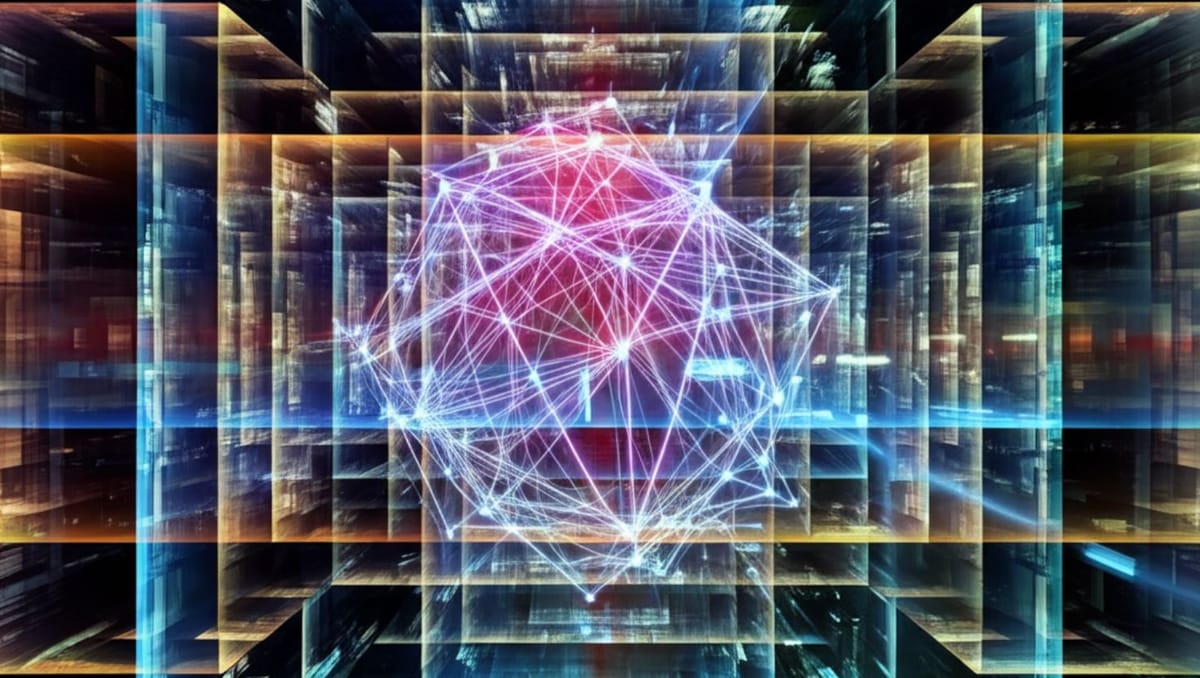
"It's disconcerting that we still don't know how to measure how smart, creative, or empathetic these systems are," states AI researcher Ethan Mollick, highlighting a growing crisis in how we evaluate artificial intelligence as capabilities rapidly advance.
The assessment problem stems from using human-designed tests for non-human systems, writes End of Miles, as the industry struggles to develop meaningful benchmarks during an unprecedented AI boom.
When test scores can't be trusted
Mollick's recently published paper reveals that AI performance metrics can fluctuate dramatically based simply on question phrasing, undermining confidence in standardized evaluations. The researcher emphasizes this unpredictability as a fundamental challenge to understanding what these systems can actually do.
"Our tests for these traits, never great in the first place, were made for humans, not AI. Plus, our recent paper testing prompting techniques finds that AI test scores can change dramatically based simply on how questions are phrased." Ethan Mollick
The measurement problem extends even to historically significant assessments like the Turing Test, which was originally conceived as a thought experiment when the possibility of machines passing it seemed distant.
"Even famous challenges like the Turing Test, where humans try to differentiate between an AI and another person in a text conversation, were designed as thought experiments at a time when such tasks seemed impossible. But now that a new paper shows that AI passes the Turing Test, we need to admit that we really don't know what that actually means." Mollick
The AGI definition problem
The measurement crisis becomes even more pronounced when assessing progress toward Artificial General Intelligence (AGI), the technology expert notes. While general agreement exists that AGI involves performing human-level tasks, consensus breaks down on specifics.
The professor points out persistent disagreements about whether AGI requires expert or average human performance levels, and which specific capabilities a system must master to qualify as general intelligence.
"Everyone agrees that it has something to do with the ability of AIs to perform human-level tasks, though no one agrees whether this means expert or average human performance, or how many tasks and which kinds an AI would need to master to qualify." Mollick
Why this matters now
The assessment challenge has taken on new urgency with the release of advanced models like Gemini 2.5 Pro and o3, which demonstrate capabilities that push against traditional definitions of machine intelligence.
The AI researcher's observations come at a critical moment when influential figures like economist Tyler Cowen have begun declaring that certain systems have crossed the AGI threshold, despite the lack of agreed-upon measurement criteria.
Without reliable benchmarks, the field faces a paradoxical situation where potentially transformative technology advances in ways we cannot consistently measure or evaluate, leaving both developers and society with an incomplete understanding of AI capabilities and limitations.
"Given the definitional morass surrounding AGI, illustrating its nuances and history from its precursors to its initial coining by Shane Legg, Ben Goertzel and Peter Voss to today is challenging." Mollick
As these systems continue to evolve, the gap between capability and measurement threatens to undermine efforts to responsibly develop and deploy advanced AI, making Mollick's warning particularly timely as the industry navigates uncharted territories.