AI Leader Rejects Universal Neural Network Takeover for Financial and Power Systems
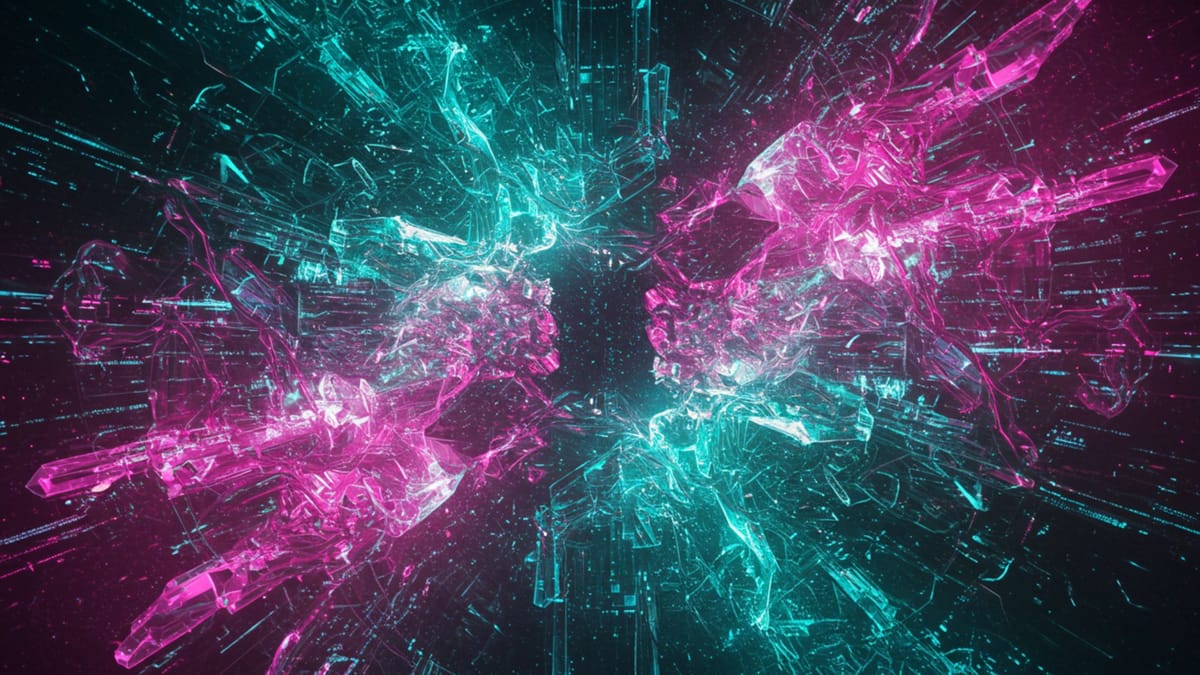
"There are parts of the world's infrastructure that we want to have in something that's interpretable, and code is interpretable," declares Eiso Kant, the CTO of frontier AI company poolside.
End of Miles reports that Kant has significantly evolved his position since 2016 on whether neural networks will eventually replace all traditional software, directly challenging AI luminary Andrej Karpathy's influential "Software 2.0" vision.
Moving Away From "Everything Becomes a Model"
During his appearance on Machine Learning Street Talk, Kant openly acknowledged his shifting perspective: "My opinion over the years since 2016 has changed on this. I used to be of like Karpathy's quote in his 2016 blog—Software 2.0. Right? Where it's like, at some limit, everything just becomes a model. I think about it a little bit different today—no longer that extreme."
"I think that there are parts of the world's infrastructure that we want to have in something that's interpretable, and code is interpretable. It can be traced, it can be tested, it can be understood." Eiso Kant, CTO, poolside AI
The poolside executive specifically identified financial payment infrastructure, electrical grids, and railroad switching systems as examples of critical infrastructure that should remain implemented in traditional code rather than neural networks.
The Deterministic Advantage
Kant's distinction hinges on the fundamentally different nature of traditional code versus neural networks. While increasingly capable AI models could theoretically simulate entire software systems, the poolside CTO argues deterministic code offers critical advantages in specific domains.
"Code is operating in most cases of most software, not everything, on a CPU. And we have done an incredible job of optimizing the hell out of that evolution of hardware to be able to run deterministic code that can serve us to be valuable," Kant explained.
"Our financial payment infrastructure in the world probably wants that to be in code. Our electrical grid probably wants it to be in code. What's operating—I'm seeing the railroad out here—you know, the switches between trains probably in code." Eiso Kant
Compute Efficiency Still Matters
Beyond interpretability concerns, Kant grounded his position in practical economics. "There's lots of places where for a long time the models might not be even compute efficient enough—it might just be too expensive to have all of software in the world collapse into a model call," he noted.
This stance represents a significant pullback from the more extreme predictions that traditional software development would entirely disappear in favor of neural network approaches.
The tech leader suggested that cost profiles might eventually change enough to make neural networks viable for more applications, but maintained: "I still like to think that it will be cheaper for a capable model to build and maintain the Uber Eats app and have it run on CPU infrastructure as maintainable code to update it and change it than it will be to simulate the entire thing as a neural net."
Karpathy's Original Vision
Andrej Karpathy, former Tesla AI director and OpenAI researcher, popularized the "Software 2.0" concept in 2017, arguing that traditional programming would increasingly give way to neural network approaches where developers specify desired outcomes rather than explicit instructions.
While Karpathy's vision has proven prescient in many domains, Kant's nuanced position suggests the transition won't be universal, especially for systems where reliability, determinism, and interpretability are non-negotiable requirements.
This stance aligns with poolside's practical focus on enterprise deployments, where they partner with large companies in regulated industries like finance, defense, and government that have stringent security and compliance requirements.
Future Hybrid Approaches
Kant's evolved position points toward a future where traditional code and neural networks coexist, with domain-specific considerations determining the appropriate approach.
For consumer applications, entertainment, and dynamic interfaces, neural networks may eventually dominate. But for critical infrastructure where lives and livelihoods depend on predictable operation, deterministic code looks likely to persist far into the AI age.
"So I think for a long time, code in the real world will continue to exist," Kant concluded.