AI Cognitive Labor Growing 25× Yearly, Outpacing Human Research 600-Fold
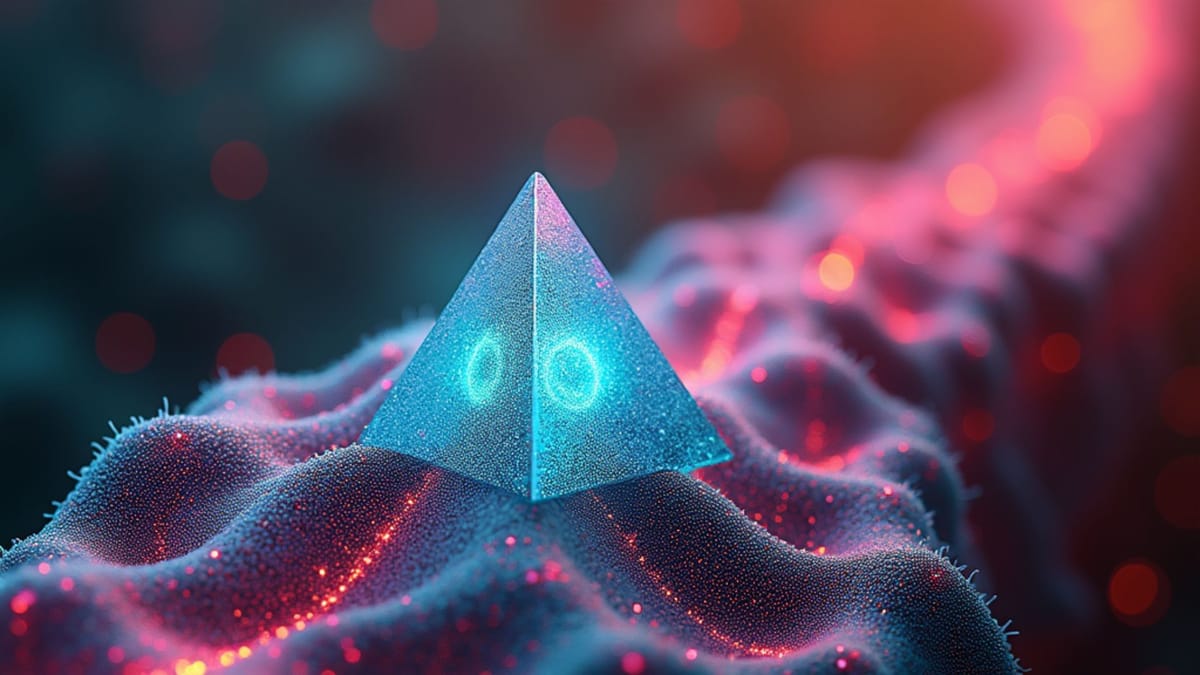
The total cognitive labor contributed by artificial intelligence systems is increasing by more than 25 times annually, vastly outpacing human cognitive growth by a factor exceeding 600, according to a comprehensive analysis published this week by Oxford philosophers.
End of Miles reports this explosive growth rate supports predictions of an imminent "intelligence explosion" – a period where AI capabilities could drive a century's worth of technological advancement in less than a decade, fundamentally reshaping civilization's trajectory.
The Mathematics of Machine Intelligence
In their paper "Preparing for the Intelligence Explosion," philosophers Fin Moorhouse and Will MacAskill present detailed calculations showing how AI capabilities are scaling at unprecedented rates across multiple dimensions.
"At the moment, the effective cognitive labour from AI models is increasing more than twenty times over, every year," the authors write. "Even if improvements slow to half their rate, AI systems could still overtake all human researchers in their contribution to technological progress, and then grow by another millionfold human researcher-equivalents within a decade." Fin Moorhouse and Will MacAskill
The Oxford academics identify multiple compounding factors driving this expansion. Inference efficiency is improving at roughly 10× per year, while inference compute – the hardware running AI systems – is increasing by approximately 2.5× annually. The multiplicative effect of these factors yields the 25× yearly growth rate in total AI research effort.
Outpacing Human Cognitive Growth
The paper quantifies, perhaps for the first time, the stark disparity between AI and human growth rates in cognitive labor. While total human research effort grows at less than 5% annually, AI cognitive capabilities are expanding more than 600 times faster.
"Current trends suggest that, over ten years, once AI reaches human parity we will get somewhere between a ten billion-fold increase in AI research capability (if compute scaling halts and even if algorithmic efficiency improvements somewhat slow down) and a hundred trillion-fold increase (if we get an aggressive software feedback loop)." The researchers
MacAskill and Moorhouse calculate that even if AI progress slows to half its current rate, it would still yield cognitive labor equivalent to millions of human researchers within years of reaching human-level capabilities in research tasks.
From Theory to Reality
The empirical evidence supporting these calculations comes from observed improvements in AI capabilities. The paper notes that in just 18 months, AI models progressed from "marginally better than random guessing" on PhD-level science questions to "outperforming PhD-level experts" on the same benchmarks.
The philosophers' analysis indicates that effective training compute – a measure combining raw computation with algorithmic improvements – is increasing by at least 10× yearly. Additional post-training enhancements provide a further 3× efficiency improvement annually.
"So, in terms of the capabilities of the best models, it's as if physical training compute is scaling more than 30× per year," the ethicists explain, noting that qualitatively new capabilities emerge from these quantitative improvements. The paper
The Oxford researchers argue that these growth rates, combined with the potential for AI to accelerate its own development through what they term a "software feedback loop," could drive a technological explosion unprecedented in human history – potentially compressing a century of progress into a decade or less.
Implications of Exponential Growth
The paper's detailed analysis frames the intelligence explosion not as speculative science fiction but as the mathematically probable outcome of current trends in AI development.
"For business to go on as usual, then current trends — in pre-training efficiency, post-training enhancements, scaling training runs and inference compute — must effectively grind to a halt," the authors conclude, arguing that without deliberate intervention, society will face a series of "grand challenges" at a pace far exceeding our institutional capacity to respond.