AI and Human Brains Process Language in Remarkably Similar Ways, Google Study Reveals
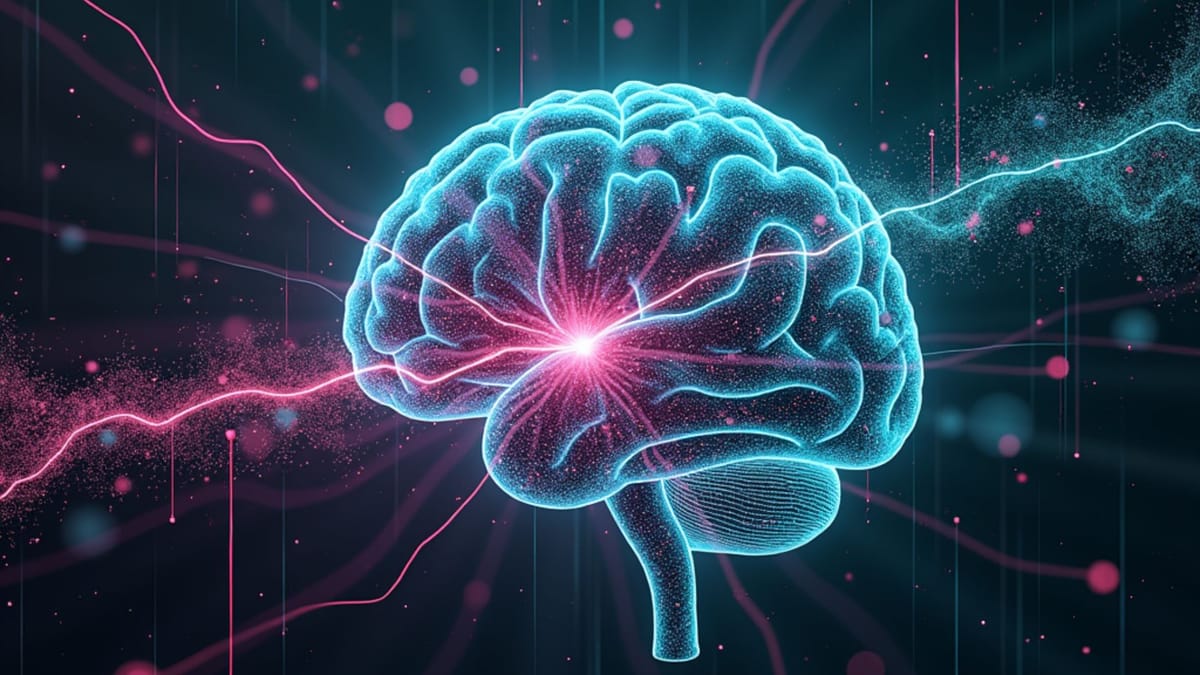
"Neural activity in the human brain aligns linearly with the internal contextual embeddings of speech and language within large language models as they process everyday conversations," reveals a groundbreaking study that fundamentally challenges our understanding of artificial intelligence and human cognition. The research demonstrates that despite different architectures, AI language models and human brains share core computational principles that were previously unrecognized.
End of Miles reports the findings represent a significant paradigm shift in how researchers understand both artificial and human intelligence, establishing one of the first direct bridges between human neural processing and AI systems.
Capturing the Brain in Conversation
The research team analyzed neural activity recorded using intracranial electrodes during spontaneous conversations, comparing these patterns with the internal representations generated by the Whisper speech-to-text model. What they found surprised even the researchers themselves.
"We sought to explore the similarities and differences in how the human brain and deep language models process natural language to achieve their remarkable capabilities," the Google Research team explained in their publication. "We demonstrate that the word-level internal embeddings generated by deep language models align with the neural activity patterns in established brain regions associated with speech comprehension and production." Google Research team
The alignment discovered was far more precise than expected. For every word heard or spoken during natural conversation, researchers could map specific neural activities to corresponding AI model processes. The team from Princeton University, NYU, and Hebrew University of Jerusalem collaborated with Google on the multi-year investigation.
The Processing Sequence Revealed
When a person listens to speech, their neural activity follows a specific sequence that mirrors AI processing stages. First, as each word is heard, speech embeddings predict cortical activity in speech areas along the brain's superior temporal gyrus. Moments later, as the listener begins decoding meaning, language embeddings predict activity in Broca's area.
Even more fascinating, the sequence reverses during speech production, with language areas activating first, followed by motor areas for articulation, and finally perceptual speech areas for self-monitoring.
"This alignment was not guaranteed — a negative result would have shown little to no correspondence between the embeddings and neural signals, indicating that the model's representations did not capture the brain's language processing mechanisms." Research publication
Why This Matters Beyond the Lab
The Princeton-affiliated researchers discovered that both the brain and AI systems use a "soft hierarchy" in neural processing, where brain regions prioritize certain aspects of language while still capturing multiple processing levels. The superior temporal gyrus prioritizes acoustic features but still captures word-level information, while language areas like the inferior frontal gyrus prioritize semantics but also register lower-level features.
These findings challenge the long-held assumption that artificial intelligence and human cognition operate on fundamentally different principles. Instead, the research suggests that deep learning models could offer a new computational framework for understanding the brain's neural code based on principles of statistical learning and optimization.
The AI specialists acknowledge significant differences remain between artificial and biological systems. Unlike transformer-based AI, which processes hundreds of words simultaneously, human language areas analyze language serially, word by word, recurrently, and temporally.
Moving forward, the research team plans to create innovative, biologically inspired artificial neural networks with improved capabilities by adapting architecture and training protocols to better match human cognitive processes — potentially leading to the next major breakthrough in artificial intelligence design.